Exploring Google DeepMind: Transforming AI Landscape
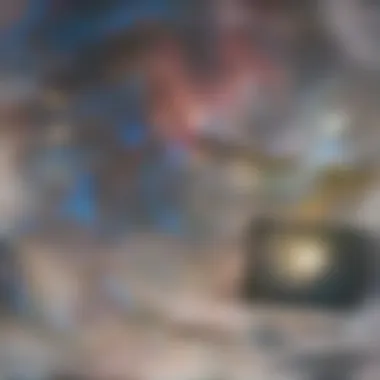
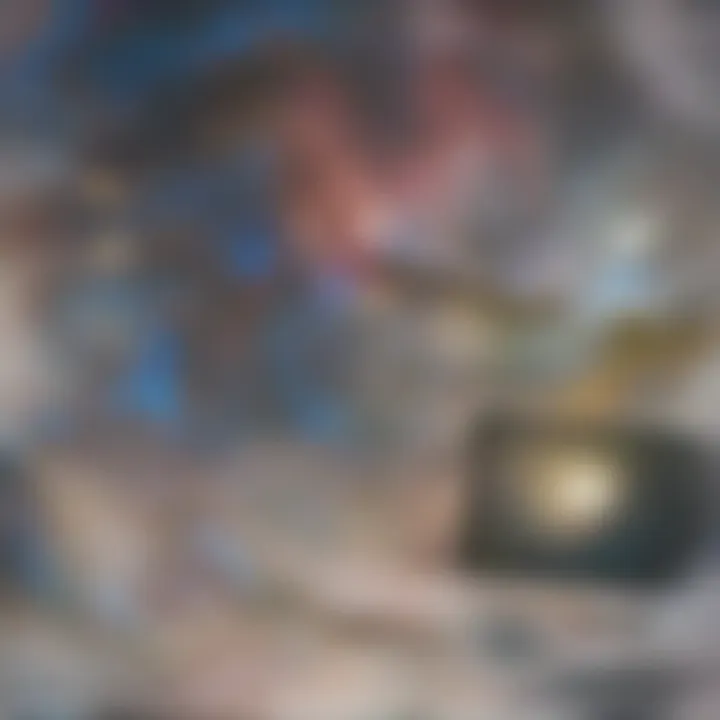
Intro
In the realm of artificial intelligence, Google DeepMind stands as a leading force. The organization has profoundly impacted how AI is developed and applied across various fields. Exploring its innovative contributions reveals not just technological advancements, but also the ethical complexities intertwined with these breakthroughs.
This article will provide an expansive insight into the foundational elements of Google DeepMind. We will examine its revolutionary technologies, the notable achievements that have marked its journey, and the implications of its work in a broader societal context. Furthermore, we will address significant ethical considerations and the future trajectory of deep learning and AI research.
Research Overview
Summary of Key Findings
Google DeepMind has demonstrated remarkable capabilities in several areas of artificial intelligence. Key findings include:
- Breakthroughs in Reinforcement Learning: Applications like AlphaGo demonstrate the power of reinforcement learning.
- Advancements in Healthcare: Projects such as DeepMind Health aim to improve medical diagnostics and treatment.
- Applications in Energy Efficiency: Innovations help reduce energy consumption in data centers.
These findings illustrate the multifaceted nature of DeepMind's work, as it intersects with various sectors.
Background and Context
Founded in 2010 and acquired by Google in 2015, DeepMind emerged with a vision to explore the potential of AI. The organization initially focused on games, which provided a testing ground for its algorithms. Over time, it expanded its reach into practical applications, including healthcare and energy management. Understanding this trajectory is crucial in grasping the significance of their innovations today.
Methodology
Experimental Design
DeepMind employs a variety of experimental frameworks to support its research. This includes simulations in controlled environments to improve AI learning outcomes. Games such as Go, chess, and video games serve as platforms for testing and refining algorithms.
Data Collection Techniques
Data collection at DeepMind is rigorous and multi-faceted. The organization utilizes:
- Public Datasets: Leveraging pre-existing data for model training.
- Collaborative Projects: Partnering with institutions like Moorfields Eye Hospital to collect applicable data in healthcare settings.
This approach to data collection ensures that models are well-informed and grounded in real-world application.
Preface to Google DeepMind
Google DeepMind stands at the forefront of artificial intelligence research, committed to pushing the boundaries of what AI can achieve. Understanding its evolution and impact is vital for grasping the current state and future of AI technologies. This section delves into the origins and milestones of DeepMind, offering insights into its innovative culture and foundational technologies that set the stage for its breakthroughs.
Origins and Formation
DeepMind was founded in 2010 by Demis Hassabis, Shane Legg, and Mustafa Suleyman in London. The company arose from the desire to create highly intelligent systems capable of learning and thinking like humans. Its formation was influenced by prior research in neuroscience, cognitive sciences, and machine learning. The foundational approach combined elements from various existing technologies to foster a new era in AI development.
As DeepMind grew, it emerged as a significant player in the tech landscape. The team consisted of experts from diverse backgrounds, including neuroscience and software engineering. Their collective insights laid a robust framework for investigating complex problems and pushing the boundaries of traditional machine-learning methods.
Acquisition by Google
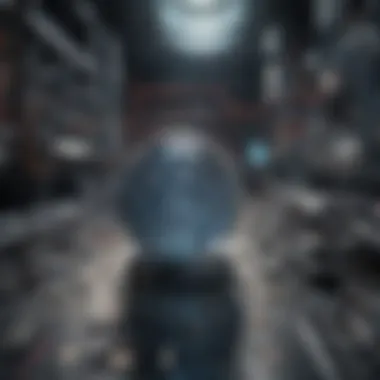
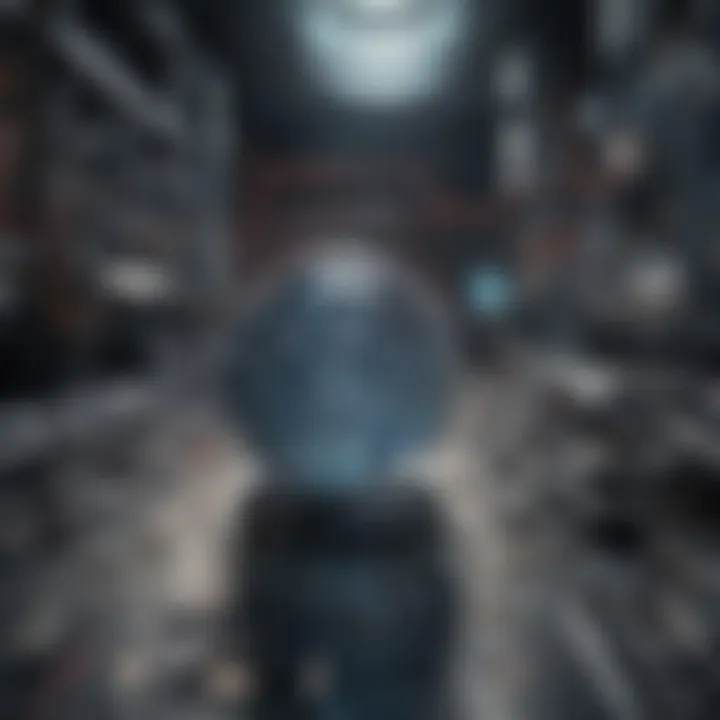
In 2014, Google acquired DeepMind for a sum reportedly around $500 million. This acquisition marked a pivotal moment not only for DeepMind but also for the broader tech industry, signaling a shift in how large tech firms viewed artificial intelligence. Post-acquisition, DeepMind benefitted from Google’s expansive resources, infrastructure, and global reach.
Integration within Google allowed DeepMind to refine its research capabilities significantly. Leveraging access to vast datasets and advanced computing power transformed its projects, enabling it to iterate rapidly on AI models. Google also provided a platform for DeepMind’s applications, using them across various products like Google Assistant and other Google services.
In summary, the history of Google DeepMind is a narrative of ambition, innovative spirit, and strategic collaboration. Understanding these origins is crucial for appreciating its subsequent advancements and the role it plays in shaping the AI of the future.
Key Technologies Developed
Google DeepMind has significantly influenced the landscape of artificial intelligence through its groundbreaking technologies. The advancements made in the field are not merely incremental; they mark a paradigm shift in how AI can be applied across various sectors. Through innovative methodologies, DeepMind has created a robust framework for understanding complex behaviors and generating predictive models. Each technological stride is not only about improving performance but also about broadening the scope of problems that AI can tackle effectively.
Reinforcement Learning Mechanisms
Reinforcement learning (RL) stands as one of the core pillars of DeepMind's technological advancements. It is built on the principle that agents learn to make decisions by interacting with their environment. Unlike traditional supervised learning, where a model learns from labeled data, RL agents operate in a trial-and-error manner. They receive rewards or penalties based on their actions, thus optimizing their strategies over time.
The applications of reinforcement learning are vast. For example, AlphaGo, DeepMind's famous chess-playing AI, utilized RL to master the complexities of the game, learning from millions of simulations. The importance of this technology cannot be understated; it opens pathways for AI in sectors such as robotics, finance, and healthcare, enabling adaptive and intelligent decision-making systems that continuously improve.
Neural Networks Innovations
DeepMind has also made remarkable strides in neural network architectures. Innovations in this space have led to enhanced model performance and flexibility. One notable development is the use of deep learning algorithms that mimic the human brain's structures. These neural networks can model complex relationships in data that previous technologies struggled to capture.
An example includes convolutional neural networks (CNNs), which DeepMind has implemented in image recognition tasks. The ability of these networks to extract features from images has had profound implications in computer vision. Furthermore, recurrent neural networks (RNNs) have enabled advancements in sequence prediction, aiding in natural language processing tasks and time-series forecasting.
Generative Models and Simulation
Generative models are another significant area of exploration for DeepMind, focusing on creating new data samples from existing dataset distributions. They have implications in various domains, including art, music, and scientific simulations. Generative Adversarial Networks (GANs) represent one such advancement, allowing models to generate synthetic data indistinguishable from real-world data.
This capability has transformative potential, particularly in training AI systems where data may be scarce or difficult to collect. For example, simulating protein folding processes can lead to breakthroughs in drug discovery and healthcare-related research, as it allows scientists to explore complex biochemical structures rapidly.
"DeepMind's innovations in generative models could redefine how artificial intelligence is used in creative and scientific fields."
Major Breakthroughs
The significance of exploring the breakthroughs made by Google DeepMind lies in understanding how these advancements have propelled artificial intelligence to new frontiers. DeepMind has transformed the landscape of AI through its innovative applications, leading to tangible benefits across diverse sectors such as healthcare, gaming, and scientific research. Each breakthrough represents a milestone that not only highlights the technical prowess of the organization but also opens pathways for future exploration and implementation of AI technologies.
AlphaGo and Game Strategies
AlphaGo is perhaps one of the most notable achievements of Google DeepMind. This AI program became the first to defeat a human world champion in the complex board game, Go. The implications of this victory extend far beyond gaming. AlphaGo utilized a combination of deep reinforcement learning and neural networks to master strategies that were previously believed to be intuitive to human players. The algorithms learned from a multitude of games, improving progressively by analyzing previous moves and outcomes.
The victory over top players demonstrated the capabilities of AI in strategic thinking. This accomplishment challenges the traditional view of intelligence, suggesting that machines can perform tasks once thought to be solely human domains. The methodologies employed in AlphaGo have since influenced other fields. Techniques from its learning structure have found applications in logistics, finance, and even real-time strategy in other games. Thus, AlphaGo represents a paradigm shift in how we view problem-solving in complex environments.
AlphaFold and Protein Folding
AlphaFold represents another monumental breakthrough within the realm of AI, specifically in the field of biology. The challenge of protein folding has puzzled scientists for decades. Proteins play crucial roles in various biological functions, and understanding their shapes is vital for advancements in medicine and biotechnology. AlphaFold utilizes advanced neural networks to predict protein structures with unprecedented accuracy.
The accuracy achieved by AlphaFold can lead to significant improvements in drug discovery and development. By understanding how proteins fold, researchers can identify potential therapeutic targets more effectively. The application of AlphaFold not only accelerates scientific discovery but also serves as a foundation for future innovations in genomics and personalized medicine. Its release to the scientific community underscores DeepMind's commitment to collaboration and the pursuit of knowledge.
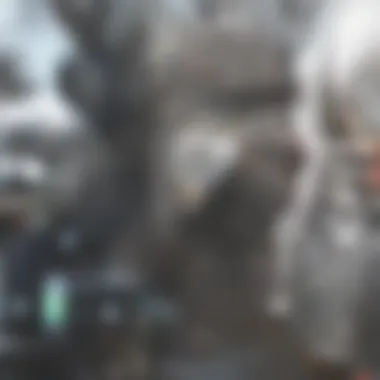
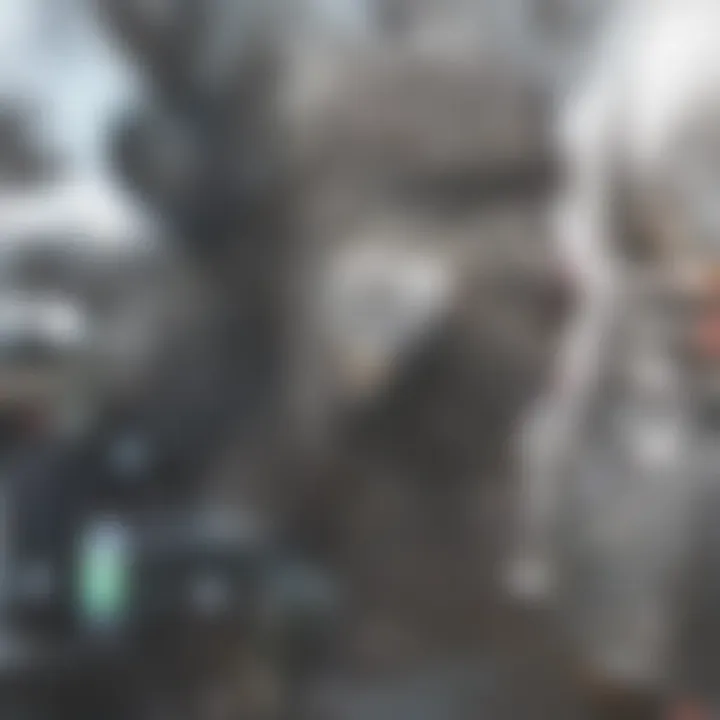
Research in Healthcare Applications
DeepMind's contributions extend into healthcare, where the organization has focused on leveraging AI for improving patient outcomes. Research initiatives such as those targeting eye diseases demonstrate the practical benefits of AI in diagnosing conditions faster and more accurately than traditional methods.
For example, the system developed to analyze retinal scans can detect signs of diseases like diabetic retinopathy. This capability is critical as it allows for earlier intervention, potentially saving sight for millions of individuals. Moreover, partnerships with healthcare providers have facilitated the integration of DeepMind's algorithms into existing medical workflows. This collaborative approach amplifies the impact of their research, creating a more informed healthcare system.
Impact on Various Domains
The influence of Google DeepMind extends far beyond technical achievements in artificial intelligence. Its work has implications across multiple fields, reshaping industries and enhancing existing processes. Understanding these impacts not only highlights the potential of AI but also emphasizes the need for balanced integration of technology into society. This section explores significant advancements in three key areas: natural language processing, robotics, and climate science.
Advancements in Natural Language Processing
Natural language processing (NLP) has seen profound advancements due to DeepMind’s innovations. Through the application of deep learning techniques, systems are now more capable of understanding and processing human language. This development has improved machine translation, sentiment analysis, and chatbots, enabling these systems to engage in more human-like conversations.
One of the standout contributions from DeepMind is the development of Transformers, a model architecture that processes data sequentially while maintaining context. This technology allows algorithms to grasp subtle meanings behind words and phrases, accommodating complexities like idioms and cultural references. As a result, NLP applications are increasingly used in sectors such as customer service, education, and content creation.
The impact of improved NLP capabilities can also be measured in accessibility. Enhanced translation services facilitate communication across linguistic barriers, promoting inclusivity and understanding on a global scale.
Enhancements in Robotics
DeepMind's influence on robotics is another pivotal area. By integrating AI with robotic systems, they have enabled machines to learn tasks through observation and practice. This process, known as imitation learning, allows robots to adapt to variable environments without extensive pre-programming. Consequently, robots can perform complex tasks in manufacturing, logistics, and healthcare, driving efficiency and productivity.
Moreover, leveraging reinforcement learning has empowered robots to refine their skills autonomously. For example, DeepMind's work in teaching robots how to manipulate objects showcases how machines can learn through trial and error, which is essential for applications requiring flexibility and responsiveness.
Applications in Climate Science
The intersection of AI and climate science represents a critical frontier for DeepMind's work. Their algorithms analyze vast datasets from environmental sensors, improving our understanding of climate patterns and predicting future trends. This capability is essential in developing strategies to combat climate change and mitigate its impact on vulnerable ecosystems.
DeepMind's approach to data analysis can enhance climate models, leading to more accurate simulations of weather patterns and ecological changes. These advancements assist researchers in crafting informed interventions to preserve biodiversity and manage natural resources more effectively.
Furthermore, the collaboration between AI researchers and climate scientists underscores how technology can serve as a tool for sustainability. Initiatives rooted in AI enable better resource management, optimizing energy usage and reducing waste in various sectors.
As DeepMind continues to push the boundaries of AI, its influence is expected to resonate across even more domains, raising important questions regarding its responsible use.
In summary, Google DeepMind's contributions significantly impact natural language processing, robotics, and climate science. These advancements not only facilitate greater efficiency but also foster innovative approaches to longstanding challenges in society. As AI continues to evolve, its role in shaping the future across these fields remains critical.
Challenges Faced by DeepMind
As Google DeepMind continues to push the boundaries of artificial intelligence, it encounters several noteworthy challenges. Addressing these obstacles is essential not only for the advancement of DeepMind’s innovations but also for the broader implications that these technologies hold for society. Understanding the challenges faced enables researchers and professionals to comprehend the current limitations while fostering an informed dialogue around AI's future. Through effective problem-solving, DeepMind can further refine its technologies and ensure responsible development in the AI field.
Technical Barriers
DeepMind's pursuit of cutting-edge technology is often hindered by technical barriers. These issues can range from computational limits to data availability and integration. One significant challenge lies in the need for massive datasets to train complex models effectively. While advancements in data collection exist, ensuring the quality and diversity of this data remains a concern. Poor quality data can lead to biased AI models, which in turn affects the reliability of the outcomes. Furthermore, the training of sophisticated models requires significant computational resources. This leads to extended training times, increasing operational costs and limiting accessibility to smaller research entities.
Moreover, even with adequate resources, achieving real-time processing and deployment of AI models presents another technical hurdle. Real-world applications often demand immediate responses, which current models struggle to meet consistently. These limitations highlight the necessity for ongoing innovation in algorithms and infrastructure to maintain DeepMind's competitive edge.
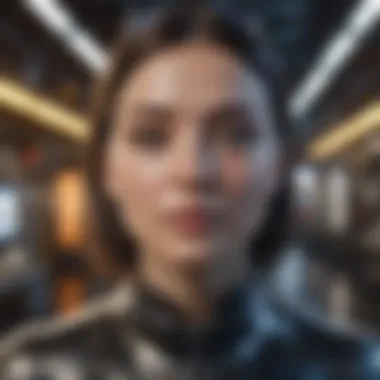
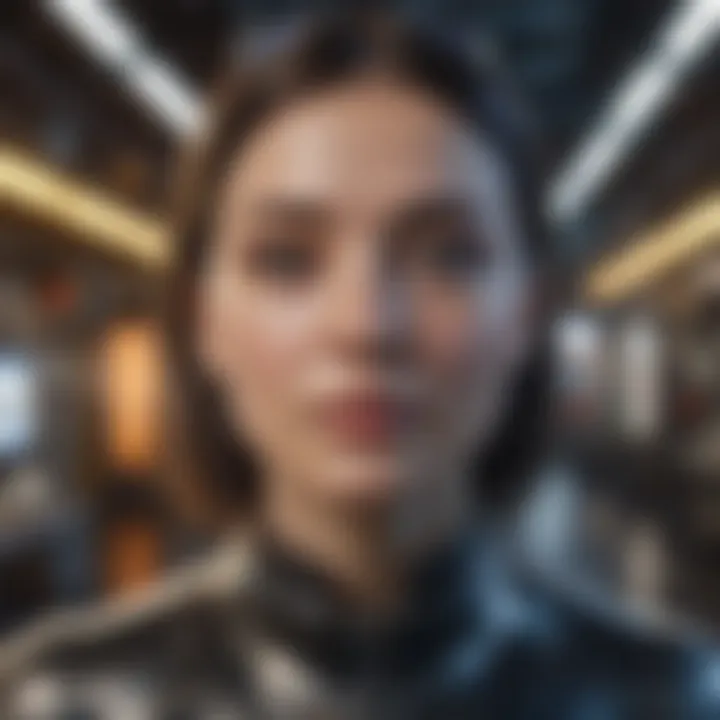
Ethical and Regulatory Considerations
Given the significant impacts of DeepMind's technologies, ethical and regulatory considerations are paramount. As AI systems become integrated into various sectors—including healthcare, finance, and public safety—the need for ethical guidelines intensifies. Concerned parties must consider how AI may affect human autonomy, privacy, and overall societal well-being.
DeepMind faces the challenge of ensuring that its technologies comply with existing regulations while lobbying for a framework that can adapt to the rapid evolution of AI. The lack of uniform regulations across different regions complicates this landscape further. For instance, the EU’s General Data Protection Regulation (GDPR) demands strict data privacy measures, which may conflict with the data-driven nature of AI development.
Furthermore, the ethical implications of AI decisions pose dilemmas that require careful consideration. For example, the use of AI in healthcare decisions raises questions about accountability. If an AI system makes an erroneous diagnosis, who is responsible? As DeepMind navigates these ethical waters, it continues to work towards creating transparent systems that maintain public trust.
"To foster widespread acceptance, developers must prioritize transparency and understanding in AI technologies."
Overall, addressing these technical barriers and ethical considerations is essential for DeepMind. As it confronts these challenges, the organization not only works to refine its technologies but also lays the groundwork for responsible AI applications in the future.
Future Prospects of DeepMind
The future of DeepMind holds significant promise. Understanding these prospects is crucial in recognizing the potential advancements in artificial intelligence and their implications on society. As technology rapidly evolves, DeepMind's continued research initiatives will likely play a pivotal role in shaping the landscape of AI. The organization is positioned not just at the forefront of AI development, but also in tackling some of the grand challenges of our time, such as healthcare, sustainability, and understanding complex data.
Potential Research Directions
DeepMind has several avenues for future research that could broaden the horizons of its contributions. These include:
- Healthcare Innovation: Building on successes like AlphaFold, DeepMind could enhance personalized medicine through advanced predictive modeling and diagnostics.
- Environmental Sustainability: Utilizing AI to optimize energy efficiency and resource management can have far-reaching benefits for climate science.
- Natural Language Understanding: DeepMind may explore deeper nuances in language understanding, improving communications between humans and AI.
- Ethical AI Frameworks: As AI systems proliferate, establishing ethical guidelines and interpreting AI decision-making processes becomes imperative.
This direction can lead to innovations that enhance work processes, improve decision making, and even democratize access to information.
Integration with Broader AI Initiatives
The integration of DeepMind’s research with broader AI initiatives is essential for fostering collaboration and societal progress. By establishing partnerships with major technology firms, academic institutions, and global agencies, DeepMind can amplify its impact. Potential areas of collaborative focus include:
- Open AI Ecosystems: Sharing insights and datasets can accelerate learning across the AI field.
- Collaborative Healthcare Projects: Partnering with organizations like NHS to apply AI in real-world scenarios further validates findings.
- Cross-disciplinary Research: Working alongside experts in fields such as neuroscience or psychology can enrich AI development and enable more humane interactions.
This integration can result in more robust AI technologies, driving mutual benefits among the various stakeholders involved.
"The future of AI is not merely about creating more capable systems; it involves creating systems that are responsible and beneficial for society."
In summary, the future prospects of DeepMind underline a commitment to both advancing technology and addressing ethical implications. As AI continues to evolve, the road ahead for DeepMind is one filled with opportunities and challenges that require thoughtful consideration.
Culmination
In this final segment, we reflect on the significance of Google DeepMind's journey and its impact on the landscape of artificial intelligence. The insights garnered from this article demonstrate how the organization's pioneering efforts have reshaped the fundamental understanding and application of AI technologies across numerous fields.
Summary of Key Contributions
Google DeepMind has made remarkable strides in AI, particularly through its key innovations. Some of the major contributions include:
- Reinforcement Learning: Techniques that enable AI to learn from its environment through trial and error, leading to groundbreaking applications in various domains.
- AlphaFold: A monumental achievement in predicting protein structures, which holds profound implications for biology and medicine.
- Natural Language Processing: Enhanced models that improve machine understanding of human language, facilitating better interaction with users.
Each innovation has not only advanced the field but has also opened new pathways for research and development. The continuous integration of AI in sectors such as healthcare, climate science, and robotics speaks volumes about DeepMind's influence.
Final Thoughts on AI Revolution
As we conclude, it’s essential to consider how Google DeepMind fits into the broader narrative of the AI revolution. The organization stands at the intersection of technology and ethics. While the advancements present undeniable benefits, they also come with dilemmas that require careful consideration. Understanding the implications of these technologies may help bridge the gap between innovation and responsibility.
Ultimately, as artificial intelligence continues to evolve, it is initiatives like those from Google DeepMind that will shape the future of this field. The journey is far from over, and ongoing research promises even more transformative solutions in the years to come.