Unveiling Lung Cancer Image Datasets and Their Impact
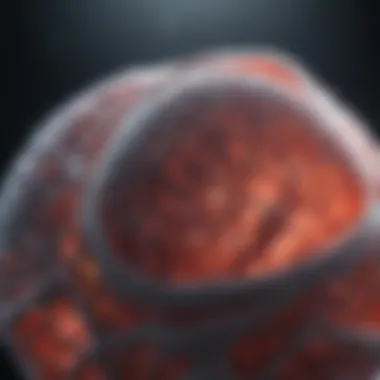
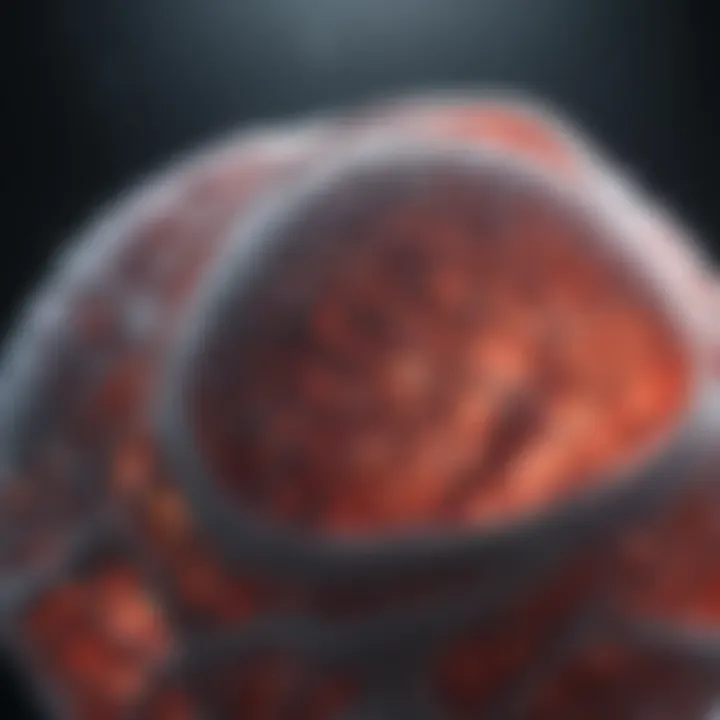
Research Overview
Lung cancer is a significant global health challenge, remaining one of the top causes of cancer-related deaths. Current advancements in imaging technologies, such as high-resolution CT scans and MRI, coupled with machine learning techniques, offer new avenues for improving diagnostic precision and treatment methods. The enhancement of these imaging technologies has been pivotal in creating vast datasets that contribute to understanding and combating lung cancer. This section will summarize key findings and present the relevant background context regarding lung cancer image datasets.
Summary of Key Findings
Research has increasingly highlighted the multifaceted role of image datasets in lung cancer studies. For example, in recent studies, datasets have been used not only to detect cancer but also to evaluate treatment effectiveness. Some key findings include:
- Image datasets improve detection rates of lung nodules, allowing for earlier intervention.
- Machine learning models trained on these datasets show significant promise in classifying cancer types and predicting outcomes.
- Further research into these datasets can guide the personalization of treatment plans based on individual patient characteristics.
Background and Context
Understanding the context of lung cancer image datasets is essential. The historical development of imaging technologies has enabled the creation of extensive datasets. Before the advent of advanced imaging techniques, lung cancer diagnosis heavily relied on less precise methods. Today, systems like the Lung Image Database Consortium (LIDC) and the National Lung Screening Trial (NLST) provide comprehensive repositories of imaging data. These datasets include diverse cases, which aids in training robust artificial intelligence models. Accessibility to such information has increased collaboration among researchers, promoting the sharing of knowledge and techniques essential in the fight against lung cancer.
Methodology
Investigating lung cancer image datasets requires a thorough understanding of the methodologies employed in their creation and application. This section will discuss experimental design and data collection techniques.
Experimental Design
The design of experiments using lung cancer image datasets often follows a structured approach. Key aspects of this design include:
- Defining clear objectives, such as demonstrating the efficacy of a particular imaging technique or algorithm.
- Selecting instruments and methodologies that align with research goals, ensuring data collected is relevant and of high quality.
- Implementing systematic protocols for analyzing images, which helps maintain consistency across studies.
Data Collection Techniques
Collecting data for lung cancer studies can take various forms, and each technique presents its own set of challenges and opportunities.
- Source Acquisition: Data may be acquired from hospitals or medical institutions, often requiring compliance with ethical regulations and patient confidentiality.
- Annotation: Radiologists typically annotate images, marking regions of interest. This process is vital for providing context and supporting data-driven research.
- Standardization: It is essential to standardize imaging protocols to ensure that images are comparable. Variability in image quality can significantly affect research outcomes.
"The utilization of standardized imaging protocols can greatly enhance the consistency and reliability of lung cancer studies."
By thoroughly examining these aspects, researchers can effectively leverage lung cancer image datasets to contribute to advancements in diagnostics and treatment planning.
Prelude to Lung Cancer Image Datasets
Lung cancer continues to be one of the most diagnosed cancers worldwide, making the quest for accurate diagnosis and effective treatment crucial. One pivotal element that plays a significant role in such efforts is the image datasets used for lung cancer research. Understanding these datasets provides valuable insights for numerous professionals, including clinicians, researchers, and students.
The utilization of imaging technologies presents an array of benefits, primarily enhancing diagnostic performance and guiding therapeutic decisions. Moreover, as challenges with lung cancer diagnosis persist, the relevance of high-quality image datasets cannot be overlooked. They serve as foundational components for the development of advanced machine learning algorithms one can apply for predictive analysis in clinical settings.
In this article, we will explore important aspects of lung cancer image datasets. We will discuss their vital role in diagnosis, the various imaging techniques employed, and the public and private datasets available. Further sections will delve into data collection methods, potential challenges, and the innovative applications of artificial intelligence in this ever-evolving field.
Importance of Imaging in Lung Cancer Diagnosis
Imaging is central to lung cancer diagnosis as it allows clinicians to visualize tumors and assess their characteristics. Early detection through imaging can lead to better treatment outcomes. High-resolution images provide the data needed for accurate tumor staging and planning interventions.
Furthermore, imaging technologies facilitate ongoing monitoring during treatment to evaluate response and adjust strategies accordingly. This capability is particularly significant given the complexity of lung cancer, the various subtypes, and how they respond differently to therapies. The integration of imaging data with clinical information can lead to more personalized treatment approaches, ultimately improving patient outcomes.
Overview of Common Imaging Techniques
Different imaging modalities are employed in lung cancer diagnosis, each offering unique advantages. A brief overview is as follows:
X-rays
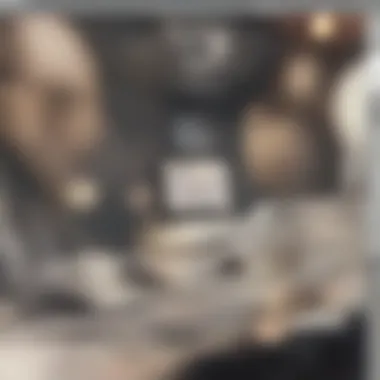
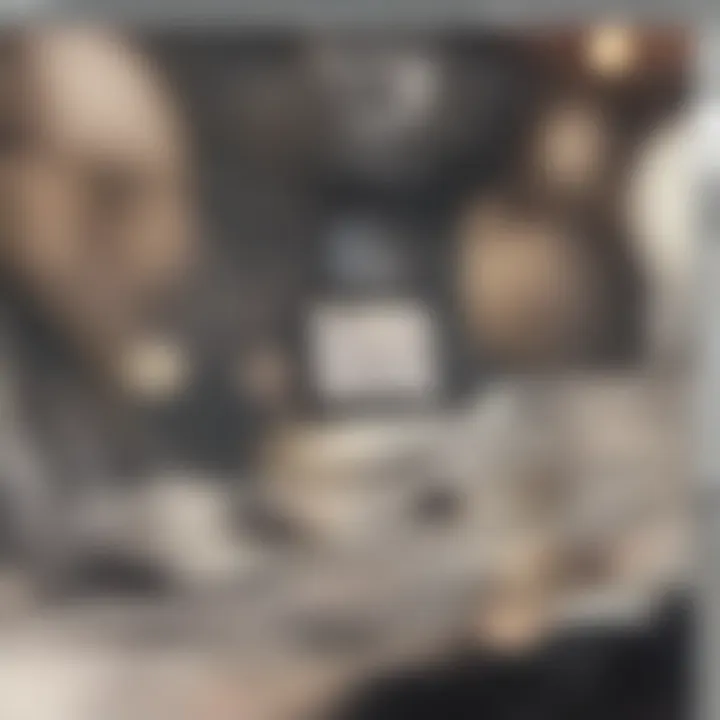
X-rays are often the first step in lung cancer diagnosis. Their primary advantage lies in their availability and speed. They are effective in identifying abnormalities such as masses or nodules. However, they provide limited detail compared to other imaging methods, which may necessitate further evaluation with advanced techniques.
CT scans
CT scans offer a more detailed view than X-rays. They produce cross-sectional images of the chest, which help accurately identify the size, shape, and location of tumors. This allows for better assessment of tumor spread. While highly beneficial, CT scans expose patients to more radiation, which is a consideration in managing patient care.
MRI
MRIs differ from CT scans as they use magnetic fields rather than radiation. They are particularly useful in evaluating the involvement of surrounding tissues and can provide excellent contrast in soft tissue imaging. However, MRIs are generally more time-consuming and expensive, limiting their widespread use for lung cancer diagnosis.
PET scans
PET scans are crucial for understanding metabolic activities within the body. They are often used to gauge whether a tumor is active and to assist in staging cancer. While very informative, PET scans typically require the injection of a radioactive tracer and may not be suitable for all patients, particularly those with specific health issues.
These various imaging techniques complement each other, providing a comprehensive foundation for diagnosing and managing lung cancer more effectively, enhancing the overall understanding required in data collection and usage.
Types of Image Datasets in Lung Cancer Research
The classification of image datasets in lung cancer research is crucial, providing the foundation for advancements in diagnostic practices and treatment strategies. These datasets can be broadly categorized into publicly available datasets and private or clinical datasets. Publicly available datasets are often utilized for educational and research purposes, enabling researchers to build models with less friction. In contrast, private datasets lead to more focused and specific insights affecting patient care.
The use of different datasets facilitates a deeper understanding of the disease by offering a variety of images and accompanying clinical data. This variety not only enhances statistical power in research but also supports the validation of machine learning models that aim to predict outcomes related to lung cancer. Therefore, comprehending the types of image datasets is paramount for researchers, educators, and medical professionals looking to contribute meaningfully to the field.
Publicly Available Datasets
Publicly available datasets play an essential role in lung cancer image research. They provide researchers access to extensive collections of imaging data, often annotated, which can enhance learning algorithms and promote collaboration.
LUNA16
LUNA16, the Lung Nodule Analysis 2016 Challenge, serves as a significant resource. It is composed of computed tomography (CT) scans, specifically focusing on the detection of lung nodules. A key characteristic of LUNA16 is its large volume of annotations linked to several scans. The dataset provides researchers a solid ground for developing algorithms to identify potential lung cancers early on.
This dataset is particularly beneficial for students and professionals aiming to test the performance of their algorithms in a standardized format. However, one limitation is that LUNA16 contains primarily the nodule images, which may not offer the full clinical context often required when diagnosing patients.
NSCLC Radiogenomics
NSCLC Radiogenomics dataset bridges the gap between imaging and genomics. By linking radiological data with genomic profiles of non-small cell lung cancer patients, this dataset enables researchers to look at cancer through a comprehensive lens. One of its notable aspects is its incorporation of both imaging features and the molecular characteristics of tumors. This dual approach is compelling because it addresses personalized medicine, providing insights into how imaging can guide therapeutic decisions. Nevertheless, the complexity and size of the dataset may deter new researchers who might find it challenging to navigate the integrative aspects of imaging and genetic data.
Lung Image Database Consortium ()
The Lung Image Database Consortium (LIDC) focuses on pulmonary images and their utility in semi-automated systems. A primary strength of this dataset lies in its extensive labeling for nodules, lymph nodes, and other associated structures, making it a valuable asset for researchers in lung cancer assessment. The LIDC dataset is well regarded for its systematic approach to image annotation, allowing for reproducibility in research. However, given its scope, it can be time-consuming to process and interpret the vast amount of data encapsulated within the dataset, especially for those less experienced.
Private and Clinical Datasets
Private and clinical datasets often offer richer and more nuanced insights compared to their public counterparts. They are generally collected within medical settings and typically feature high-quality images along with detailed patient histories.
These datasets may include data from specific hospitals or research projects, ensuring that the information is tailored and directly applicable to clinical scenarios. The drawback here is that access is often restricted due to privacy concerns and ethical regulations.
Nonetheless, they hold the potential for more meaningful research outcomes, providing insights that could ultimately influence clinical practice.
Data Collection Methods for Lung Cancer Images
The methods employed in collecting images for lung cancer research are critical. These methodologies establish the foundation for generating reliable and valid datasets. A rigorous approach to data collection ensures high-quality images, which are essential for accurate analysis and interpretation. There are varying methods for gathering images, including scanning protocols, equipment used, and the patient populations involved. Each of these elements contributes not only to the technical aspects of the collected data but also affects its applicability in real-world clinical settings.
Ethical Considerations and Regulations
The ethical dimensions of data collection in lung cancer imaging are significant. When obtaining medical images, consent from patients is paramount. It is critical to ensure that patients are fully informed about how their images will be used, particularly in research contexts. Regulations such as the Health Insurance Portability and Accountability Act (HIPAA) in the United States stipulate how patient data, including images, should be handled and protected. Adhering to ethical guidelines ensures that patient privacy is maintained and fosters trust between researchers and participants.
Metadata Associated with Imaging Studies
Metadata plays an essential role in the context of lung cancer imaging studies. It refers to supplementary data that provides a framework for understanding medical images. Metadata typically includes information on the imaging process, patient demographics, and clinical outcomes.
Patient demographics
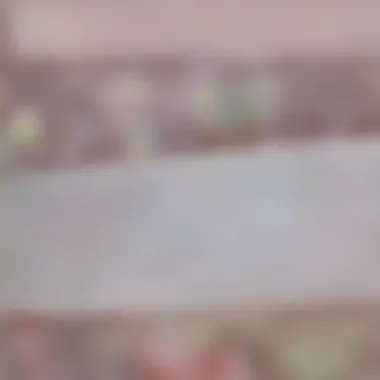
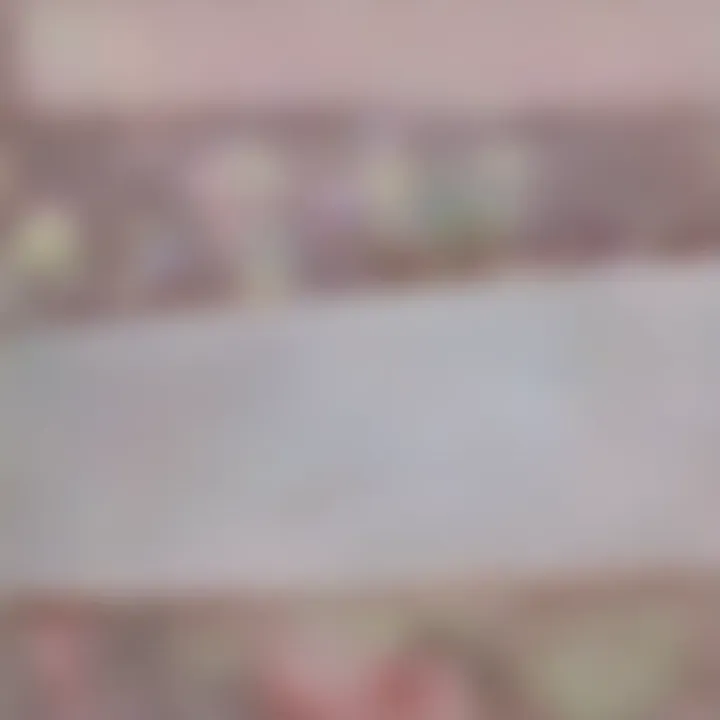
Patient demographics pertain to various factors such as age, gender, ethnicity, and medical history. Collecting this data is crucial because it allows for stratification of imaging results, leading to more tailored analyses. For instance, understanding age differences in tumor presentations can influence diagnostic strategies. A key characteristic of patient demographics is their variability, which can reflect diverse biological responses to lung cancer. This diversity is a beneficial element in developing generalized treatment strategies that are both effective and adaptable. However, it also presents challenges, as demographic data can sometimes be incomplete, which may introduce biases in research conclusions.
Clinical outcomes
Clinical outcomes refer to measurable impacts of treatments and interventions over time. This metadata is fundamentally important for understanding the effectiveness of various imaging techniques in diagnosing lung cancer. A fundamental characteristic of clinical outcomes is their direct correlation with patient survival and quality of life. Including this data allows researchers to determine the predictive value of imaging modalities in terms of patient prognosis. A unique feature of assessing clinical outcomes is the potential for long-term studies, which can provide insights into the effectiveness of early interventions. However, tracking clinical outcomes over time can be logistically challenging and demands ongoing coordination within healthcare settings.
In summary, the methodologies for data collection in lung cancer research are multifaceted and involve ethical regulations and detailed metadata, both of which ensure the integrity of research outputs.
Challenges in Lung Cancer Imaging Dataset Utilization
Lung cancer imaging datasets play a crucial role in enhancing diagnostic processes and treatment strategies. However, the utilization of these datasets presents several challenges that need careful consideration. Addressing these challenges is essential for improving the overall effectiveness of lung cancer research and clinical applications. Here, we will explore two prominent challenges: data quality and standardization issues, and large-scale data management. Understanding these intricacies is vital for creating reliable and effective solutions in pulmonary oncology.
Data Quality and Standardization Issues
Data quality is a fundamental aspect of any imaging dataset. In lung cancer, it directly influences the ability of researchers to draw meaningful conclusions and develop robust AI models. Variability in imaging techniques, machine settings, and patient conditions often leads to inconsistencies in image quality.
Moreover, the lack of standardization across datasets can complicate data integration from multiple sources. For instance, a CT scan from one institution may differ significantly from another due to the equipment used or the protocols followed. Standardization efforts, such as establishing universal protocols for imaging acquisitions and annotations, are crucial. This can help ensure that the data being analyzed is comparable across different studies, enhancing the validity of findings.
Additionally, an emphasis on automated image quality assessment can help identify and rectify inferior images before they enter analytical pipelines. The integration of machine learning algorithms for this purpose offers a promising path to improve data quality and reproducibility.
Issues with Large-scale Data Management
As the volume of imaging data continues to grow, managing large-scale datasets has become a significant challenge. The sheer size of lung cancer imaging datasets poses unique hurdles. This includes ensuring efficient storage solutions, facilitating rapid access, and providing seamless data sharing among researchers.
With data coming from various sources, it becomes imperative to have a well-thought-out database architecture. It needs to support various data formats and types while also enabling rapid retrieval. Poor data management can lead to delays in research progress and hinder collaborative efforts.
Furthermore, maintaining privacy and security standards is critical, especially when handling sensitive patient information. Compliance with regulations like HIPAA and GDPR must be at the forefront of data management strategies. Researchers must utilize encryption and access controls to safeguard data integrity and patient confidentiality.
In summary, the challenges in lung cancer imaging dataset utilization are multifaceted and require comprehensive strategies for resolution. By addressing data quality and standardization issues, as well as managing large-scale data effectively, researchers can enhance the utility of imaging datasets for advancing lung cancer diagnosis and treatment.
Artificial Intelligence and Machine Learning in Lung Cancer Imaging
Artificial intelligence (AI) and machine learning (ML) are changing the landscape of lung cancer imaging, offering transformative potential in diagnostic processes and therapeutic interventions. The integration of AI and ML into lung cancer imaging allows for enhanced image analysis, automating tasks that were once manual, and providing insights that can lead to earlier detection and more personalized treatment plans. This section delves into specific elements of deep learning techniques and their impact on diagnostic accuracy.
Application of Deep Learning Techniques
Deep learning, a subset of machine learning, employs neural networks with many layers to analyze data representations. In lung cancer imaging, these techniques have shown great promise in identifying patterns that may be overlooked by human observers. For instance, convolutional neural networks (CNNs) have been effective in distinguishing between benign and malignant nodules in CT scans. These models are trained on large datasets, which helps improve their performance by learning from a vast array of examples.
The application of deep learning techniques also extends to the enhancement of imaging modalities. For example, generative adversarial networks (GANs) can produce high-quality synthetic images that can be used to augment existing datasets. This can be particularly beneficial in cases where data is scarce, thus enhancing the robustness of training sets for deep learning models. The consistency these techniques bring to image analysis increases the ability to deploy reliable and reproducible diagnostic tools.
Impact of AI on Diagnostic Accuracy
The introduction of AI in lung cancer imaging significantly affects diagnostic accuracy. AI algorithms can analyze images at a speed and precision level that surpasses human analysts. This capability is essential, particularly given the fast-paced nature of clinical settings where time is crucial. Studies have demonstrated that AI systems can achieve similar or even better performance than radiologists in certain diagnostic tasks, such as detecting lung cancer from chest X-rays or CT scans.
Moreover, AI reduces human error and biases by providing a standardized assessment approach. The algorithms focus entirely on the data, which can help minimize variations in interpretation caused by human factors such as fatigue or varying levels of experience. While AI cannot replace human expertise, it serves as a valuable tool that can enhance the efficacy and reliability of diagnosis.
As research continues, the collaboration between AI systems and healthcare professionals is expected to flourish. This partnership aims to improve clinical outcomes and streamline diagnostic workflows in lung cancer care.
Moving forward, the integration of AI in clinical practice requires careful consideration of ethical implications and regulatory standards to ensure safe and effective implementations. The ongoing advancements in machine learning and AI technologies signal a promising future in lung cancer imaging, paving the way for improved patient care and outcomes.
Case Studies in Lung Cancer Image Analysis
Understanding the application of imaging techniques in lung cancer diagnosis and treatment can be significantly enhanced through specific case studies. These studies exemplify how AI and machine learning models successfully operate within clinical environments. They showcase both the opportunities and limitations faced in translating advanced technological solutions to practical scenarios, therefore illustrating the relevance of image datasets in lung cancer research.
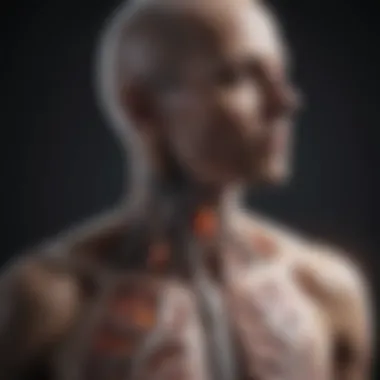
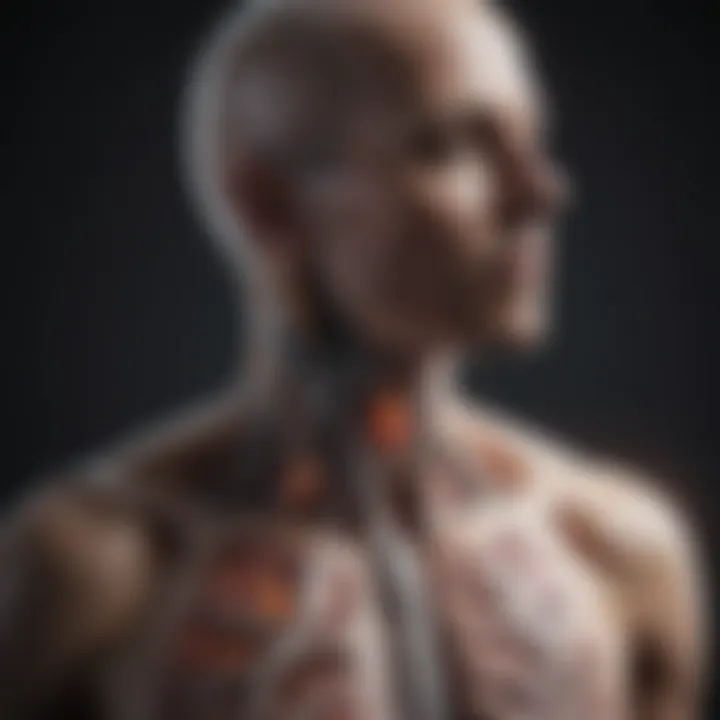
Successful Implementation of AI Models
The integration of AI models in lung cancer diagnostics has shown promising results across various case studies. For example, research conducted on the LUNA16 dataset revealed that deep learning networks could achieve high accuracy in detecting lung nodules in CT scans. This achievement is not only a testament to the power of AI but also highlights the importance of high-quality image datasets.
The ability of these models to differentiate between benign and malignant nodules significantly enhances early diagnosis, which is crucial for improving patient outcomes. Furthermore, the deployment of models such as convolutional neural networks (CNNs) in clinical settings demonstrates how automated systems can process vast amounts of imaging data swiftly, yielding quick insights for radiologists.
Key benefits observed in these studies include:
- Improved Detection Rates: AI-driven systems can identify subtle differences in imaging that might be overlooked by the human eye.
- Consistent Analysis: Algorithms provide consistent results without fatigue, which is essential in high-pressure environments.
- Support for Radiologists: By automating preliminary readings, radiologists can focus on more complex cases, thus optimizing workflow in clinical settings.
Challenges Faced in Clinical Settings
Despite the positive outcomes witnessed in case studies, the utilization of AI models also encounters significant challenges in clinical settings. One major hurdle lies in the integration of AI tools with existing healthcare infrastructure. Many hospitals and clinics are still reliant on traditional systems that may not be compatible with advanced technologies.
Additionally, data privacy and ethical issues pose another set of challenges. Handling sensitive patient information demands strict adherence to regulations, which can complicate data sharing for model training.
Moreover, varied image quality and inconsistency across datasets complicate the training process for AI models. For instance, when AI models trained on high-quality images are exposed to lower-quality images in a clinical setting, their performance can decrease sharply, leading to misdiagnosis or overlooked cases.
Important considerations include:
- Need for Standardization: Consistent image quality across datasets can greatly improve the efficacy of AI models.
- Training on Diverse Datasets: To enhance generalization, AI models must be trained on a wide range of imaging data attuned to various populations and equipment.
In summary, while case studies reveal the transformative potential of AI models in lung cancer image analysis, they also underline the necessity for collaboration between technologists and healthcare professionals to overcome the impediments to fully realizing this potential. By addressing these challenges, future research can pave the way for more effective diagnostic tools and better patient outcomes.
Future Directions in Lung Cancer Imaging Datasets
The future of lung cancer imaging datasets is poised for significant advancements. These developments will not only enhance the diagnostic landscape but will also drive innovations in treatment approaches. With the continuous evolution of technology and analytical methodologies, embracing these future directions is essential for improving patient outcomes.
Integration of Multi-modal Data
Multi-modal data integration refers to the combination of diverse data types, including imaging, genomics, and clinical records. This approach is crucial for providing a holistic view of lung cancer. By analyzing multiple sources of data, researchers can uncover richer insights into tumor behavior and treatment responses. This integration supports the following advantages:
- Enhanced Diagnosis: Holistic insights lead to more accurate diagnoses by correlating imaging patterns with genomic phenotypes.
- Improved Research Outcomes: A multi-faceted dataset opens up avenues for advanced studies, including predictive modeling and clinical trials.
- Comprehensive Patient Profiles: Understanding the patient on multiple levels, from molecular details to imaging findings, aids in tailored treatment.
However, challenges such as data standardization and compatibility persist, requiring efforts in data harmonization and advanced algorithms.
Potential for Personalized Medicine
Personalized medicine aims to tailor treatment based on individual patient characteristics. Lung cancer imaging datasets play a pivotal role in enabling this approach. With enhanced imaging techniques and machine learning algorithms, healthcare providers can identify optimal treatment strategies tailored to a patient's unique tumor profile. The potential for personalized medicine is promising because it:
- Increases Treatment Efficacy: Targeted therapies can be developed that align with specific tumor characteristics, potentially leading to better responses.
- Reduces Adverse Effects: By choosing therapies based on individual needs, unnecessary side effects can be minimized, improving overall quality of life.
- Facilitates Continuous Monitoring: Enhanced imaging data allows for ongoing tracking of tumor response to treatment, ensuring timely modifications when necessary.
"The integration of imaging datasets into personalized medicine could reshape the treatment landscape for lung cancer, emphasizing the need for continued advancements in this field."
End
In closing, the exploration of lung cancer image datasets marks a significant advancement in the fight against this formidable disease. The integration of imaging technology and machine learning methodologies fosters a landscape where diagnostic precision can be greatly improved. Key elements such as the standardization of data, and ethical considerations surrounding patient information, are essential for creating effective analysis frameworks. This article highlights not only the pressing challenges faced in utilizing existing datasets but also underscores the benefits that can be reaped through thoughtful application of technology and data science in clinical settings.
The importance of analyzing various image datasets adds depth to our understanding of lung cancer. Research, paired with the correct technological tools, can lead to better diagnostic procedures and innovative treatment strategies.
Key Takeaways
- Significance of Data: Image datasets serve as the backbone for research advancements in lung cancer.
- Interdisciplinary Approach: Collaboration among clinicians, data scientists, and researchers enhances the utility of imaging data.
- Ethical Practices: Ensuring ethical handling of data is crucial for maintaining patient trust and scientific integrity.
- Technological Integration: The role of AI and machine learning is paramount for improving accuracy in diagnosis and treatment regimens.
Call for Collaborative Research Efforts
To fully realize the potential of lung cancer image datasets, a call for collaborative research efforts is paramount. This encompasses not just the sharing of data but also pooling expertise from various scientific domains. Collaborative efforts could include:
- Establishing joint ventures between public health institutions and universities.
- Encouraging partnerships with AI technology firms to develop novel solutions for data analysis.
- Fostering open discussions and workshops to enable knowledge exchange among researchers.
Creating a unified platform for collaboration can significantly accelerate progress in lung cancer research, leading to tangible outcomes that can improve patient care and outcomes. Taking these collaborative steps will ultimately enhance our understanding and treatment of lung cancer.