The Most Advanced AI in the World: A Comprehensive Exploration
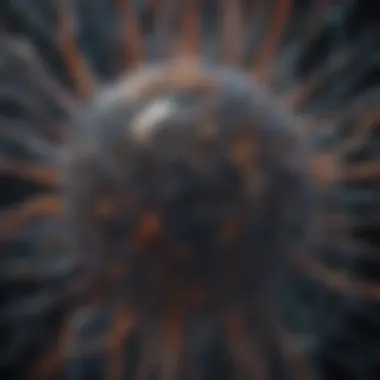
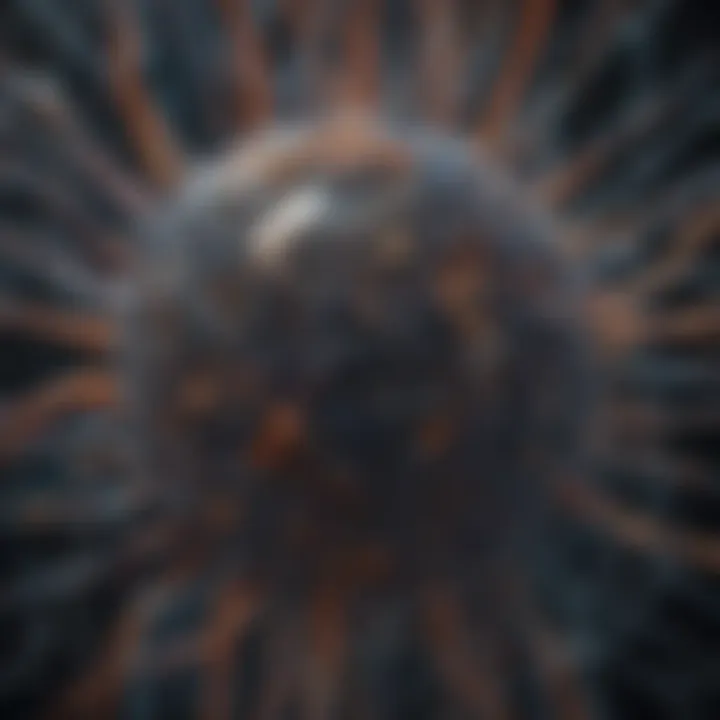
Intro
Artificial Intelligence (AI) has raised significant interest in recent years. This field is no longer just a theoretical concept, but rather a complex system that is actively reshaping multiple sectors. From healthcare to finance, advanced AI systems are no longer confined to academic research. They have transcended into tools that drive real-time decision-making, improving efficiency and outcomes.
Understanding the nuances of these sophisticated technologies requires a structured exploration. The following sections will delve into the engineering behind leading AI models, their operational capabilities, and the ethical dilemmas they pose. This exploration aims to provide an analytical lens through which students, researchers, and professionals can examine the intersections of AI technology and society.
In a world increasingly influenced by algorithms, the knowledge of what drives these systems becomes essential. This includes not only their architectural frameworks but also the broader implications of their deployment in everyday life. By critically assessing these elements, we will engage with a topic that is not only relevant but crucial for the future.
Defining Advanced AI
Defining advanced AI is crucial for understanding the complexities and capabilities of artificial intelligence. This section examines how these definitions shape perceptions and guide research in the field. It sets the stage for a comprehensive exploration of AI's capabilities and implications.
Evolution of AI Concepts
The journey of artificial intelligence spans several decades, beginning with simple rule-based systems. Early AI was limited in scope, executing predefined tasks with little flexibility. As technology advanced, researchers developed new concepts such as machine learning and neural networks, greatly expanding AI's potential. Machine learning allowed systems to learn from data, improving their performance without explicit programming.
The introduction of deep learning, a subset of machine learning, further transformed AI. This approach mimics human brain structures, using multi-layered neural networks to process information. Over time, AI systems evolved from performing specific tasks to exhibiting capabilities such as natural language processing and image recognition. Such advancements have initiated discussions on what it means for an AI to be considered advanced.
The concept of advanced AI now encompasses systems that can learn autonomously, adapt to new information, and even understand complex concepts. This evolution highlights the necessity to constantly redefine what we consider "advanced" in the context of ongoing technological progress.
Criteria for Advancement
In evaluating AI technology, several criteria help clarify what constitutes advancement:
- Learning Capacity: An advanced AI should have the ability to learn from data and improve over time without human intervention.
- Adaptive Algorithms: The flexibility to adjust methods based on input is vital. Advanced AI can change strategy when faced with new challenges.
- Autonomy: While many systems require human oversight, advanced AI can operate independently within set parameters, making decisions on their own.
- Complex Problem Solving: Advanced AI must tackle sophisticated problems that require a multi-faceted understanding and reasoning.
- Human-Like Interaction: The ability to interact with users in natural language and understand context is central to determining advancement.
Understanding these criteria aids researchers and industry leaders in identifying and fostering the development of systems that push the boundaries of artificial intelligence.
"The definition of advanced AI should adapt, reflecting changes in technology and society's expectations."
In summary, defining advanced AI is about grasping not only its current state but also the trajectory of its evolution. The criteria for advancement assist in recognizing how these technologies can integrate into various aspects of life, shaping our future.
Current Leaders in AI Technology
The landscape of artificial intelligence is rapidly evolving, with a few entities taking the lead in developing and deploying cutting-edge technologies. Understanding the current leaders in AI is essential, as these companies not only define the technological frontier but also shape the future of industries worldwide. They set benchmarks for innovation, challenge ethical considerations, and impact societal transformation. Their advancements often dictate market trends and have a direct correlation with how AI systems will be integrated into everyday life.
Overview of Key Players
The dominant forces in AI technology include Google, Microsoft, OpenAI, and IBM. Each company contributes uniquely to the AI ecosystem and pushes the envelope in terms of capability and application.
- Google: Through its Google AI division, it focuses heavily on machine learning and deep learning. Technologies ranging from Google Assistant to their groundbreaking work on Fiber Networks reflect the range and ambition of their AI initiatives.
- Microsoft: Their Azure AI platform empowers businesses with tools for integrating machine learning into applications. Furthermore, Microsoft invests in research that explores responsible AI usage and extensive partnerships to enhance commercial applications.
- OpenAI: Known for its significant advancements in natural language processing, including the development of the GPT-series models, OpenAI aims to advance AI toward a higher level of intelligence. Their commitment to ensuring safety and ethical deployment is also noteworthy.
- IBM: The IBM Watson system is a flagship AI offering that excels in data analysis, and it's widely used across healthcare and finance sectors. With their emphasis on explainable AI, IBM addresses accountability and transparency in AI algorithms.
Understanding the strengths and objectives of these key players reveals how collaboration and competition drive innovation. Each company represents different aspects of AI, from research, ethical considerations, to practical applications.
Comparative Analysis of Systems
When analyzing the AI systems of these leaders, several factors come into play. Each organization's parameters, from algorithms to hardware, affect performance and applicability. Here’s a closer look at some of these critical aspects:
- Performance Metrics: Companies often use distinct benchmarks to measure AI performance. Google’s TensorFlow may excel in certain computational tasks, while OpenAI’s models may lead in tasks involving natural language understanding.
- Scalability: Microsoft’s Azure allows clients to scale solutions based on needs, which is critical for dynamic businesses balancing multiple operational environments.
- Integration: IBM Watson stands out for its ability to integrate seamlessly into existing workflows. This aspect is crucial for industries that prioritize continuity while adopting new technologies.
- Ethpos and Responsibility: Different companies adopt varying approaches towards ethical considerations. OpenAI’s philosophy centers around responsible AI development, ensuring that their technologies benefit humanity.
"AI technology's evolution is not just about advancement but also about ensuring its ethical implications are thoroughly examined to benefit society as a whole."
Architectural Innovations
In the realm of artificial intelligence, architectural innovations are pivotal as they form the backbone of the most advanced systems. These innovations allow AI to process information more effectively and efficiently, ultimately improving performance in various applications. By understanding the specifics of AI architecture, one can appreciate not only the complexity involved but also the potential benefits of these systems in contemporary society.
Neural Networks
Neural networks represent a fundamental component of AI architecture. Inspired by the human brain, these structures consist of interconnected layers of nodes, known as neurons, that work collaboratively to process data. The capacity of neural networks to learn from large datasets makes them particularly effective at identifying patterns and making predictions.
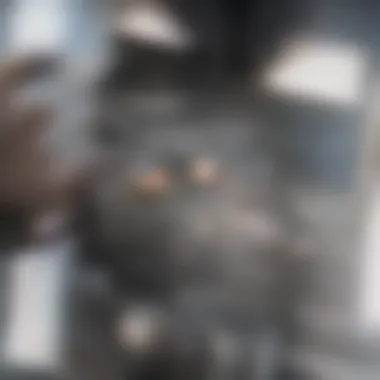
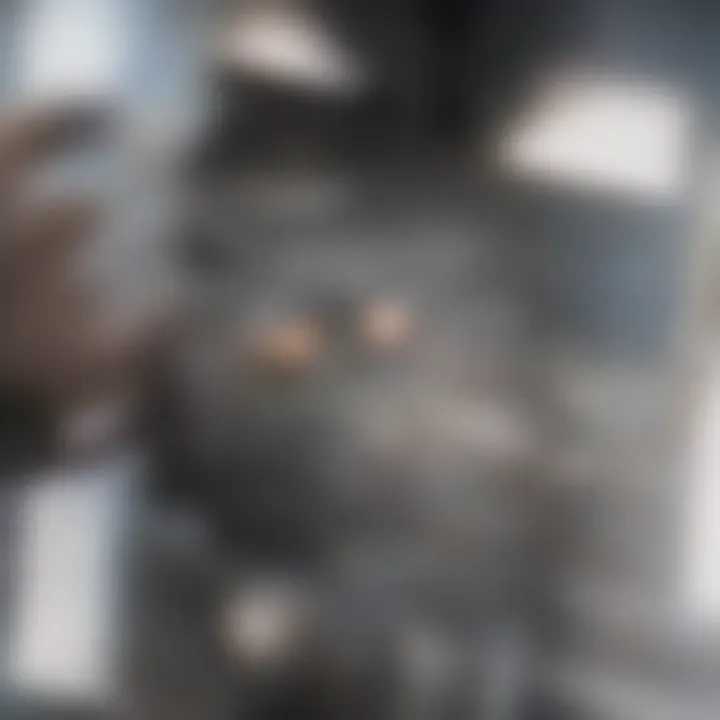
Neural networks have several advantages. They inherently handle non-linear relationships in data, which traditional programming methods often struggle with. As such, neural networks excel in fields such as image and speech recognition.
"Neural networks demonstrate a remarkable ability to approximate complex functions, making them versatile tools in AI development."
However, there are consideration to take into account. Training these networks requires substantial computational power and large amounts of labeled data. Furthermore, they are often perceived as "black boxes" since understanding how they arrive at specific conclusions can be challenging.
Deep Learning Mechanisms
Deep learning mechanisms extend the capabilities of traditional neural networks. They utilize multiple layers of neurons to extract intricate features from data, allowing for even deeper insights. This layered approach enables the model to learn hierarchically, with each layer capturing progressively more abstract representations of the input data.
Deep learning has transformed numerous industries. For instance, in healthcare, it aids in diagnosing conditions through medical imaging analysis. In autonomous vehicles, it processes sensor input to identify objects in real-time.
In essence, deep learning systems significantly outperform simpler models, especially in applications requiring vast datasets. They do, however, demand significant resources both in terms of data and computational power. This can result in increased costs and longer training times, necessitating careful project planning and resource allocation.
Natural Language Processing
Natural language processing (NLP) is an area of AI architecture focused on the interaction between computers and human language. Through NLP, machines can understand and interpret text and speech, enabling more natural interactions with users.
One major benefit of NLP is its capacity to analyze sentiment, extract information, and generate human-like responses, thereby improving customer service and interaction efficiency. Systems like OpenAI's GPT-3 reveal the profound capabilities inherent in modern NLP. Their ability to generate fluent responses based on context showcases the advancements in language models.
Nonetheless, NLP presents particular challenges. Contextual understanding can vary, resulting in misinterpretations. Additionally, the training datasets may carry biases, leading to fairness concerns and potential misapplication in sensitive scenarios.
Applications of Advanced AI
The significance of advanced artificial intelligence in contemporary society cannot be overstated. The potential applications of AI systems span diverse sectors, fundamentally transforming how we approach problem-solving and efficiency. By exploring these applications, we can comprehend their benefits and challenges, revealing the integral role they play in shaping modern industry and services. Each application reflects a unique intersection of technology and practical utility, demonstrating the remarkable versatility of AI systems.
Healthcare Innovations
In the realm of healthcare, AI has emerged as a pivotal force for improvement. Technologies such as machine learning and data analytics support medical professionals in diagnosing diseases with greater accuracy. For instance, AI algorithms analyze vast data sets from various sources, including patient histories and medical imaging, to identify patterns often missed by human eyes. This capability leads to early detection and timely intervention, which can save lives.
Another noteworthy advancement is the implementation of AI in personalized medicine. By leveraging genetic data, machine learning models can predict patient responses to various treatments, tailoring therapies to individual needs. This shift towards personalized healthcare enhances treatment effectiveness and minimizes side effects, providing patients with targeted approaches.
"AI's integration into healthcare is revolutionizing patient outcomes, making early interventions more reliable and personalized care feasible."
Moreover, AI-powered virtual health assistants, like chatbots, improve patient engagement by providing instant access to information and support. These systems can guide patients through their healthcare journey, from scheduling appointments to providing medication reminders, ultimately leading to improved adherence and satisfaction.
AI in Financial Services
The financial sector has also witnessed a profound transformation due to advanced AI applications. Algorithms are deployed in areas such as fraud detection and risk assessment, allowing institutions to analyze transactions in real-time. By identifying unusual patterns, financial organizations can quickly address potential fraud cases, thereby protecting consumers and minimizing losses.
Additionally, AI enhances customer service through automated chatbot systems that handle inquiries and transactions. These bots are available 24/7, offering users quick responses and reducing the strain on human agents. Investment strategies have also benefitted from AI. Algorithms can analyze market trends, news, and social media sentiment to inform trading decisions, providing investors with a competitive edge.
AI in Transportation
The transportation sector is undergoing significant changes through advanced AI technologies. Autonomous vehicles are at the forefront of this shift, promising safer and more efficient road travel. By utilizing AI-powered sensors and systems, these vehicles can interpret their surroundings, making decisions in real time to navigate complex environments.
AI also plays a critical role in traffic management. Intelligent systems analyze traffic patterns and adjust signal timings accordingly, improving overall flow and reducing congestion. This capability not only enhances travel times but also decreases fuel consumption and emissions.
Furthermore, AI is instrumental in logistics and supply chain management. Predictive analytics help companies forecast demand, optimizing inventory levels and delivery routes. As a result, organizations experience cost savings and improved service levels by utilizing AI for effective resource allocation.
Overall, the applications of advanced AI in healthcare, finance, and transportation illustrate its transformative potential. These technologies are not only improving efficiency and accuracy but also leading to better outcomes in various aspects of everyday life. Thorough understanding of these advancements allows for informed discussions on their implications and future possibilities.
Ethical Considerations
The rise of advanced artificial intelligence systems introduces significant ethical dilemmas that are increasingly relevant in modern discourse. Addressing these concerns is paramount not only for the integrity of AI development but also for maintaining public trust in these systems. Moreover, the ethical considerations surrounding AI influence policy-making, the direction of technological research, and the societal implications of AI applications. A deep understanding of these dynamics is essential for students, researchers, educators, and professionals working within the field.
Data Privacy Issues
Data privacy stands at the forefront of ethical considerations in advanced AI applications. As AI systems increasingly rely on vast datasets, ensuring the protection of individual privacy rights becomes critical. Unintended data breaches can lead to unauthorized access to sensitive information, exposing individuals and organizations to significant risk. The use of algorithms to analyze personal data can lead to outcomes that are not only intrusive but also discriminatory.
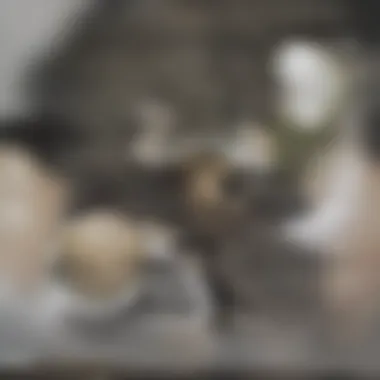
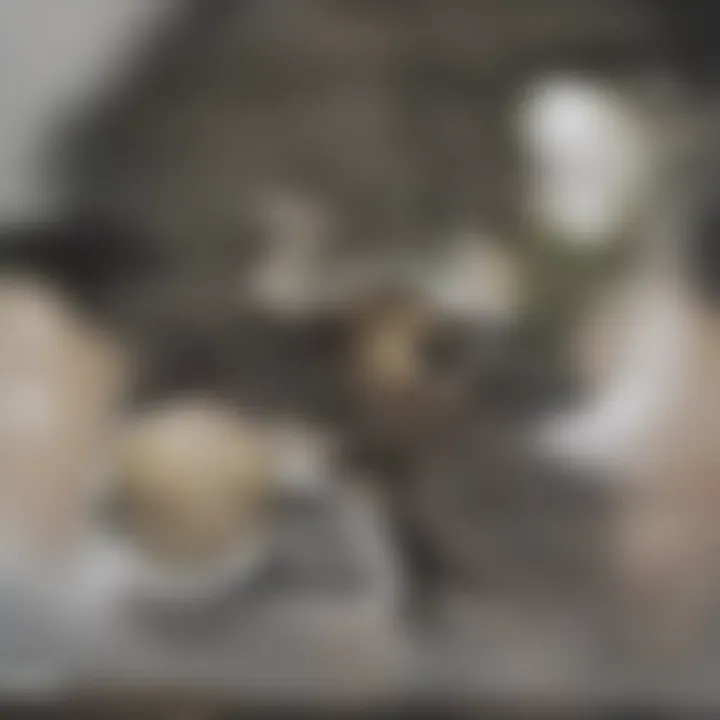
For instance, companies like Palantir Technologies utilize data collection tools that might infringe on personal privacy without consent. The balancing act between data utilization for improving AI systems and protecting user privacy is delicate. Therefore, clear regulations and ethical guidelines are essential to govern data collection practices, ensuring transparency and integrity in AI operations.
Bias and Fairness in AI
Bias in AI systems can perpetuate or exacerbate existing social inequalities. Algorithms trained on flawed or incomplete data sets can generate outputs that unfairly favor one group over another. This bias can manifest in various sectors, including hiring, law enforcement, and healthcare. The ethical implications are profound, as such biases can shape public opinion and impact lives.
Developers need to implement fairness measures during the design phase, which includes auditing algorithms for potential biases. The tools need continuous improvement, informed by diverse data inputs and inclusive practices. Organizations, including Google, have explored frameworks to enhance fairness in machine learning models. A proactive approach to bias detection can result in more equitable outcomes.
Accountability in Machine Learning
Accountability is a crucial element in the ethics of machine learning. In an environment where AI systems make decisions increasingly independently, determining accountability in the case of errors becomes complex. If an AI-driven decision leads to harm, who is responsible? The developer, the organization, or the AI itself? This ambiguity can create legal and moral dilemmas.
Establishing clear guidelines for accountability involves defining the roles of creators, operators, and users. It is vital to have processes in place for responding to unintended consequences of AI systems. Organizations must be transparent about their decision-making processes and be prepared to explain these decisions in understandable terms.
"The design and operational framework of AI should promote accountability to assure safety and fairness in application."
In summary, addressing the ethical considerations of AI—especially data privacy, bias, and accountability—requires a concerted effort from developers and organizations. This effort is essential not only for creating responsible AI systems but also for fostering public trust and ensuring that the benefits of AI advancements are distributed equitably.
Impact on Workforce Dynamics
The integration of advanced artificial intelligence systems into various sectors raises significant questions about their influence on workforce dynamics. As AI technologies evolve, they do not merely automate tasks but also reshape the skills required in the labor market. Understanding these dynamics is essential for students, researchers, educators, and professionals, as grappling with the ramifications of AI is vital for future job preparedness.
Job Displacement Concerns
One of the foremost concerns surrounding advanced AI is job displacement. As more businesses adopt AI, numerous roles, particularly those that involve repetitive or predictable tasks, are at risk. For instance, manufacturing, data entry, and even some customer service positions face the threat of automation. The World Economic Forum predicts that millions of jobs could be displaced by 2025 due to technological advancements. This scenario raises critical questions about economic stability and individual livelihoods.
While some argue that job displacement is a natural progression in technological development, others highlight the urgent need for retraining and reskilling initiatives. The automation of labor can lead to a skills gap, wherein the existing workforce lacks the necessary qualifications to transition to new roles created by AI. Addressing these concerns requires a proactive approach from educational institutions and employers to facilitate upskilling programs.
"The advancement of AI necessitates an urgent reevaluation of workforce strategies to ensure sustainable employment opportunities for all."
Transformation of Job Profiles
As advanced AI systems become more prevalent, the profiles of jobs themselves will transform. Rather than completely eliminating positions, AI often augments human roles, necessitating workers to adopt new skills. For example, in healthcare, medical professionals must learn to work alongside AI diagnostic tools. This shift demands a combination of technical proficiency and soft skills, such as communication and empathy.
Jobs are increasingly evolving from simple execution of tasks to more complex problem-solving and decision-making frameworks. This involves critical thinking and the ability to work collaboratively with AI systems. Industries are beginning to recognize the value of human-augmented intelligence, wherein AI serves as a support tool rather than a replacement.
As the landscape changes, organizations may need to restructure job roles, incorporating teams that blend AI capabilities with human insights. Not only will this enhance productivity, but it will also ensure that workers remain relevant in this rapidly changing environment.
Future Directions in AI Research
Research in artificial intelligence is continually evolving. This evolution is of immense importance to various fields, as it shapes future innovations and solutions to complex problems. Understanding future directions in AI helps organizations, researchers, and policymakers remain informed about upcoming trends. By exploring these directions, stakeholders can better prepare for ethical, social, and economic implications that advanced AI might bring.
Interdisciplinary Approaches
One significant avenue in future AI research involves interdisciplinary collaboration. AI problems often require insights from multiple fields such as computer science, biology, cognitive psychology, and sociology. Each discipline offers unique perspectives that enhance AI's capabilities. For instance, insights from neuroscience can influence deep learning algorithms, driving them closer to human-like cognitive functions.
Another notable point is the necessity for interdisciplinary training. Educating researchers and practitioners in multiple domains can create more versatile experts. This convergence allows for a range of solutions, tackling complex problems more effectively. Incorporating perspectives from diverse fields can help mitigate biases, as interdisciplinary teams are less likely to overlook significant factors affecting AI systems.
Emerging Technologies
The field of AI is witnessing the rise of several emerging technologies that promise to propel its advancements. Notable examples include quantum computing, which may significantly enhance the computational power available for deep learning. Moreover, edge computing is becoming increasingly relevant, allowing processing to occur closer to data sources, thus reducing latency and improving efficiency.
Furthermore, advancements in robotics and automation continue to reshape industries. From manufacturing to healthcare, sophisticated robots can perform tasks that were once deemed impossible. This leads to greater efficiency and innovative solutions in various sectors.
As these technologies progress, the integration of AI with Internet of Things (IoT) devices is expected to revolutionize multiple aspects of daily life. With interconnected systems, enhanced data analysis, and decision-making processes become more efficient and robust.
"The potential of advanced AI technology lies not only in its capabilities but also in how it integrates with emerging technological trends."
Global Perspectives on AI Development
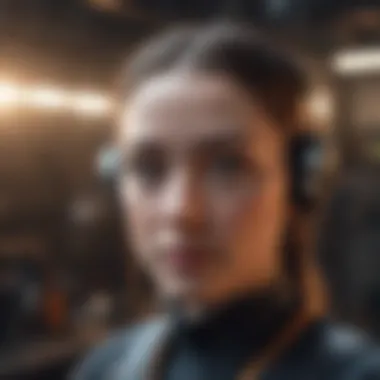
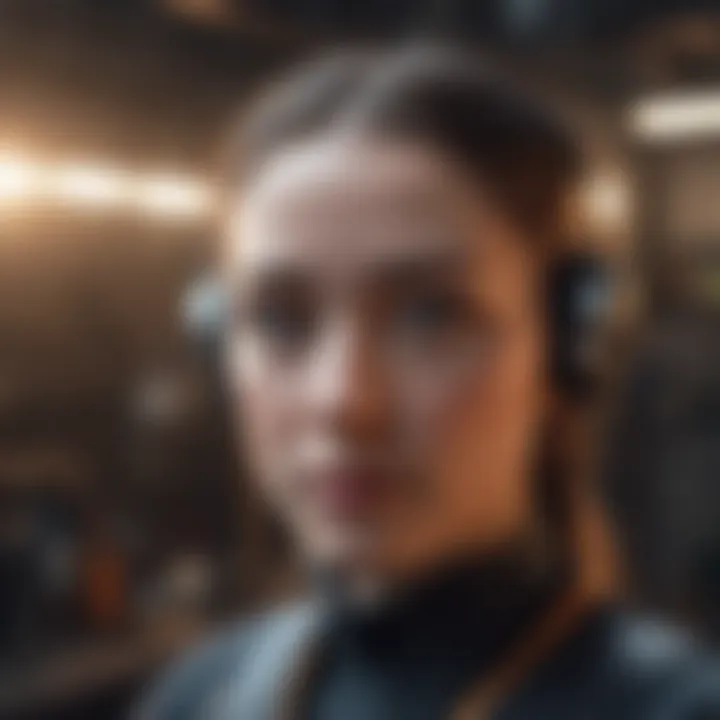
Understanding global perspectives on AI development is crucial for grasping the vast landscape of current advancements and potential trajectories. The way nations approach artificial intelligence not only reflects their economic priorities but also shapes the global digital economy. AI is not merely a technological progression; it influences social structures, educational systems, and cultural norms across different regions.
AI in Developing Nations
AI adoption in developing nations is increasingly significant. Many of these countries strive to harness AI for various sectors, such as agriculture, healthcare, and education. The potential benefits are enormous, including improved efficiency, better resource management, and increased access to information.
However, there are challenges. Often, developing nations face issues like a lack of infrastructure, limited investment in technology, and an insufficient number of skilled professionals. Addressing these barriers is essential for successful AI integration. Additionally, fostering local talent through education and training programs can strengthen a country's capability to innovate.
For instance, countries like India and Kenya are investing in AI initiatives that focus on solving local problems. In Kenya, AI technology is used to enhance agricultural productivity by providing farmers with data-driven insights into weather patterns and pest management. This demonstrates the potential of localized applications of AI technology in fostering economic growth and social development.
International Collaborations
Collaboration between nations is fundamental for advancing AI research and development. Global challenges like climate change, health crises, and food security require coordinated responses that leverage the strengths of various countries. International partnerships can facilitate knowledge sharing, resource pooling, and collaborative innovation.
Many governments and organizations are initiating joint ventures. Examples include the partnership between universities and tech companies from different countries to address AI ethics or standards. These collaborations can lead to unified approaches in regulation, which is vital when considering the global nature of AI technology.
Moreover, platforms like the Global Partnership on Artificial Intelligence emphasize the importance of AI that is responsible and inclusive. Such initiatives aim to create shared guidelines and frameworks that can help democratize access to AI technologies, benefiting all countries, regardless of development status.
"Collaboration is not just desirable; it is necessary for developing a globally responsible approach to artificial intelligence."
In summary, examining the global perspectives on AI development reveals a complex interplay between opportunity and challenge. AI in developing nations shows potential for transformative impact, while international collaboration is key to addressing shared issues regarding ethics and standards in AI deployment.
Public Perception of Advanced AI
The public perception of advanced artificial intelligence plays a significant role in its development and deployment. Understanding this perception is crucial as it can influence policy, funding, and research priorities. People’s beliefs about AI often stem from personal experiences, cultural influences, and media portrayals. Therefore, addressing public concerns is essential for fostering trust and acceptance of AI technology.
Understanding Common Misconceptions
Misconceptions about AI abound. Some individuals view AI as a near-omniscient entity that can make perfect decisions without human error. This is far from reality. Advanced AI systems like OpenAI's ChatGPT and Google's DeepMind operate within specific parameters and rely heavily on the data they are trained on. They are not infallible. Here are some common misconceptions:
- AI can think and feel like a human. In truth, AI lacks consciousness and emotions, only mimicking human-like responses based on data patterns.
- AI will inevitably replace all human jobs. While automation may disrupt certain sectors, it often creates new roles that require human oversight and creativity.
- AI can solve all complex problems. AI excels in specific tasks but struggles with nuanced issues that require emotional intelligence or ethics.
Fostering education around these misconceptions can mitigate fear and encourage responsible integration of AI technologies.
Media Representation of AI
Media plays a powerful role in shaping public perception. Films and books often dramatize AI, portraying it as either a savior or a threat. For instance, films like "Ex Machina" and "The Terminator" depict AI as entities that could potentially overpower humanity. Such narratives can contribute to anxiety and misunderstanding among the public. On the other hand, documentaries and articles that highlight real-life AI applications often present a more balanced perspective.
For instance, articles that explain how AI improves healthcare diagnostics or enhances transportation systems can inform and educate audiences of the benefits of AI without misrepresentation.
The public perception of advanced AI significantly affects its acceptance and future direction. Addressing misconceptions and promoting a realistic portrayal of AI in media are vital steps toward constructive dialogue. By educating the public and fostering a better understanding of AI's capabilities and limitations, we can enhance collaboration between developers and users, ensuring that AI technology aligns with societal needs.
Regulatory Frameworks for AI
Regulatory frameworks for artificial intelligence play a significant role in shaping the responsible development and deployment of AI systems. These frameworks are not merely documents; they represent a necessary response to the rapid evolution of AI technologies. As AI becomes integral to various sectors—like healthcare, finance, and transportation—governments and organizations must establish clear guidelines to navigate potential risks and ethical dilemmas.
The importance of regulatory frameworks can be broken down into several core elements:
- Establishing Clear Guidelines: Regulations help define acceptable practices in AI development. Clear guidelines can prevent misuse and ensure that developers adhere to ethical standards.
- Enhancing Trust: For AI technologies to be widely accepted, public trust is essential. Regulatory oversight can assure users that safety and ethical considerations are prioritized, thereby increasing public confidence.
- Promoting Innovation: While regulations can seem restrictive, they also encourage responsible innovation. They help set a baseline for safety that can drive competition and technological advancement within safer parameters.
- Encouraging Accountability: Regulations foster accountability among AI developers. They require companies to be transparent about their processes, data usage, and decision-making frameworks, which is vital for addressing biases and ensuring fairness.
- Facilitating International Cooperation: As AI is a global phenomenon, regulations can aid in standardizing practices worldwide, fostering international collaborations to address common challenges.
National AI Strategies
National AI strategies are frameworks adopted by individual countries to guide the development and regulation of AI technologies. Each national strategy typically includes objectives aligned with the nation's economic goals, social values, and ethical principles.
For instance, China's national strategic plan emphasizes technological leadership and economic growth, while the European Union advocates for a human-centric approach that prioritizes rights and ethical considerations. The presence of a well-defined national strategy is crucial because it aligns public and private interests, fosters collaboration, and provides a coherent approach toward AI governance.
International Standards and Protocols
International standards and protocols act as fences for global AI governance. They facilitate harmonious collaboration among different nations while assuring compliance with best practices. Various organizations, like the International Organization for Standardization (ISO) and the Institute of Electrical and Electronics Engineers (IEEE), are instrumental in developing these standards.
International standards serve several purposes:
- Consistency across Borders: They ensure that AI systems can operate seamlessly in various jurisdictions.
- Benchmarking: Standards provide methodologies to assess AI performance and ethical implications, encouraging best practices.
- Collaboration: They foster an environment where countries can share innovations and regulatory practices, addressing global challenges collaboratively.
"The creation of international standards for AI is crucial for realizing global cooperation and ensuring consistent and effective governance of AI technologies."