Exploring True Artificial Intelligence: Beyond the Hype
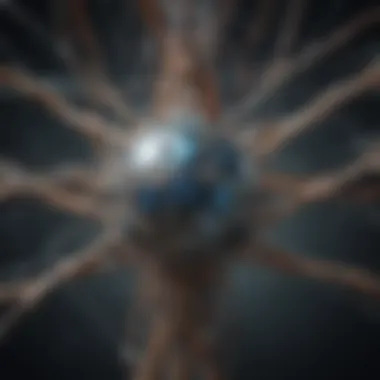
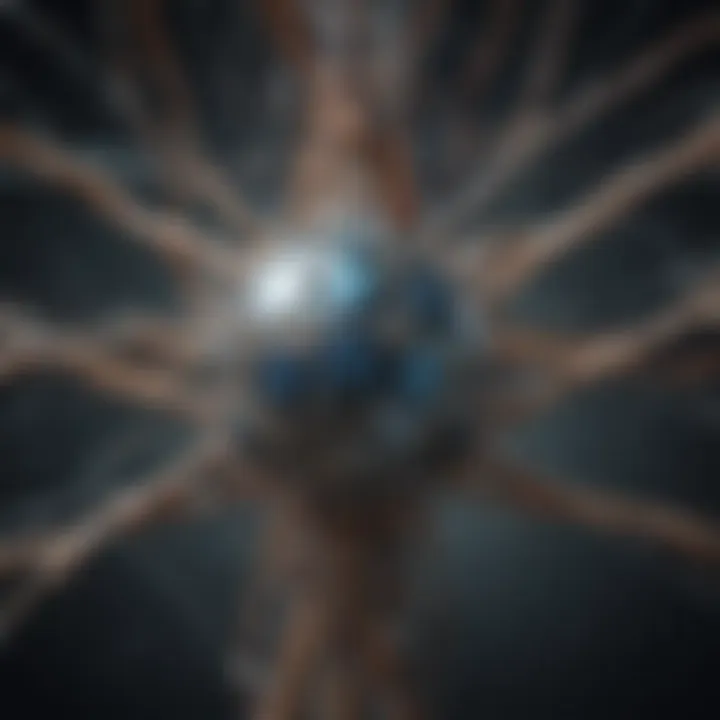
Intro
When talking about artificial intelligence, many people often wear their rose-colored glasses, dazzled by the marvels it promises. However, what lies beneath this glitzy surface is a more nuanced and multi-dimensional reality of AI, often mischaracterized as either a miracle machine or a menacing monster. Dissecting these myths and realities is critical, especially as we stand at the precipice of what many term true AI.
The exploration of true artificial intelligence isn't just an academic pursuit; it reaches into the heart of our technological and ethical frameworks. We need to dive deep into the distinctions between what's often termed narrow AI, which specializes in specific tasks, and general AI, poised to tackle any intellectual endeavor mirroring human cognitive functions. This section aims to highlight the profound implications of true AI on sectors like healthcare, education, and even the arts, while reminding us that a key aspect of this conversation is consciousness and its philosophical questions.
That said, one cannot help but wonder: with all this processing power, at what cost do we push the boundaries of AI's capabilities? The ethical quandaries are as multifaceted as the technologies themselves, demanding our undivided attention. As we embark on this journey exploring true AI, let’s unravel the complexities that underpin its evolution and potential impact on society.
Defining True Artificial Intelligence
Understanding what constitutes true artificial intelligence is pivotal in dissecting both its potential and its implications. This section aims to clarify the essence of AI, focusing on the fine line that distinguishes various iterations of technology often lumped into this category. By defining the terms clearly, readers gain a structured framework that will aid in navigating the more complex discussions throughout the article. We are not just scratching the surface here; we are diving into robust discussions that unravel the intricacies.
Distinction Between Narrow and General AI
When we talk about AI, a lot of people think of the stuff they've seen in blockbuster movies: machines that think and feel exactly like humans. However, that's a far cry from the reality we observe today. Two main forms of AI take center stage: narrow AI and general AI.
- Narrow AI refers to systems designed to perform specific tasks. Think about the recommendation algorithms used by streaming platforms like Netflix or the voice assistants in our smartphones. They can beat you at chess or recommend the next best show, but they can't engage in meaningful conversation or understand emotions like a human can.
- General AI, on the other hand, remains something of a holy grail. This form of AI would possess the ability to understand, learn, and apply knowledge much like a human. It’s not just about performing tasks; it’s about nuanced understanding and decision-making in varied contexts.
In essence, understanding this distinction is crucial for appreciating not only current AI capabilities but also the aspirations moving forward. The gulf between narrow and general AI sets the scenery for ongoing debates about functionality, ethics, and the future compatibility of machines within human frameworks.
Key Characteristics of True AI
What makes AI 'true'? Several characteristics often arise in these discussions, and acknowledging them provides clarity and focuses our aspirations around what AI could become.
- Learning Ability: True AI must exhibit an ability to learn from experience. This means that over time, systems should improve and adapt, which is more than rote memorization.
- Autonomy: The capacity to operate independently in real-world situations without constant human guidance represents a significant leap from current machine operations.
- Understanding Context: Machines should not only process data but also understand the context in which that data exists. A true AI must grasp underlying meanings, implications, and the nuances of contingencies that arise.
- Reasoning and Problem Solving: True AI would need to think critically and apply logic in its processes. It hints at a level of cognitive functioning that current AI lacks.
- Method of Interaction: How AI interacts with humans could make or break its utility. Natural and intuitive communication methods using language, tone, and gestures would enhance the human-AI dynamic.
In summary, these characteristics are not just boxes to check; they represent milestones on the path toward developing a truly intelligent system, one that offers genuine benefit rather than mere efficiency. By focusing on these characteristics, we lay down a path that future discussions can elaborate upon—a comprehensive discourse about what we strive for in true artificial intelligence.
Theoretical Foundations of AI
The backbone of any technological discipline lies in its foundational theories, and artificial intelligence (AI) is no exception. Understanding the theoretical foundations of AI helps to frame its capabilities, limitations, and future developments. It also sheds light on how AI intertwines with various fields, including cognitive science and machine learning, making this an essential area of focus. By exploring these elements, one gains insight into the core principles that guide AI innovation and implementation. Moreover, grasping these theories equips practitioners and researchers with the framework needed to tackle real-world problems effectively.
Cognitive Science and AI
Cognitive science plays a pivotal role in shaping the landscape of artificial intelligence. This interdisciplinary domain blends psychology, neuroscience, and linguistics, to name a few, to decode the mysteries of human thought processes. Understanding human cognition provides a crucial template for replicating decision-making and adaptive behaviors in machines.
One significant aspect is how insights from cognitive science contribute to developments in algorithms that mimic human learning. For instance, concepts such as perception, memory, and reasoning inform the structures behind neural networks, allowing AI systems to process information more like a human brain would.
Another noteworthy connection is found in the way AI and cognitive science interact. As AI systems evolve, they generate data that can further inform cognitive theories. This creates a cyclical enrichment of both fields, resulting in more sophisticated AI applications.
To put things into perspective, consider the way a child learns to speak. A child listens, observes, and practices words in context. Similarly, AI systems, through techniques such as supervised learning, rely on patterned data to improve their performance. Yet, this raises an intriguing question: can machines ever truly replicate the nuances of human thought?
Machine Learning Algorithms Explained
Diving into the specifics, machine learning is at the heart of AI’s functionality. But what exactly drives the learning process behind these algorithms? At its core, machine learning can be understood through several categories: supervised learning, unsupervised learning, and reinforcement learning.
- Supervised Learning: This approach relies on labeled data to guide the algorithm. An example may include a dataset of images where each picture is tagged with its respective object (like a cat or a dog). The system learns to distinguish among these objects by analyzing various features such as color and texture.
- Unsupervised Learning: Unlike its supervised counterpart, unsupervised learning deals with unlabeled data. This method aims to find hidden patterns within the dataset. Clustering algorithms, for example, group similar data points, shedding light on underlying structures without predefined labels.
- Reinforcement Learning: This category takes a different approach by allowing the machine to learn through trial and error. Much like training a dog, where you reward desired behavior, reinforcement learning uses positive feedback to encourage specific actions while penalizing incorrect ones.
The fusion of these learning strategies underpins numerous applications we encounter today—from predictive text functionalities on smartphones to advanced robotics. Thus, understanding machine learning algorithms is paramount for anyone looking to delve into the domain of AI.
In closing, the theoretical foundations of artificial intelligence not only provide a framework for understanding its development but also challenge us to think critically about what it means for machines to think, learn, and evolve. As we move forward, there's much to gain by maintaining an interdisciplinary approach that encompasses cognitive science and robust algorithm development.
Historical Context of AI Development
Understanding the historical context of AI development is crucial for grasping the depth and breadth of what true artificial intelligence embodies today. This section not only sheds light on how past breakthroughs shape current technologies, but it also provides insight into the motivations and challenges faced by pioneers. Historical milestones serve as both lessons and inspirations, informing current practices while sketching the path forward.
For instance, examining early AI experiments in the mid-20th century reveals the foundational theories and philosophies that still underpin today's innovations. It was a time when compute power was akin to a small fraction of today’s smartphones, yet the ambition to emulate human thought was already kindling a flame of curiosity. By delving into these beginnings, we can more fully appreciate the complexities of current AI debates surrounding capabilities, ethics, and future possibilities.
Milestones in AI Research
A multitude of significant milestones punctuate the history of AI research. Each of these events not only advanced the field but also provided an understanding of the technological trajectory that continues to evolve.
- The Dartmouth Conference of 1956: This event is often considered the birth of AI as a field of study. A group of researchers gathered to discuss the potential of creating "thinking machines", laying the groundwork for future exploration.
- The introduction of the perceptron in 1957: Frank Rosenblatt's perceptron was one of the first neural networks. This simple model sparked excitement but also led to disillusionment due to its limitations.
- The rise of expert systems in the 1980s: These systems revolutionized how organizations processed information. Expert systems, like MYCIN, managed to outperform humans in specialized tasks, enhancing productivity across various sectors.
- Deep learning and big data in the 2010s: The confluence of huge datasets and powerful computing unleashed a new era. Technologies such as Google’s AlphaGo showcased AI's ability to learn and adapt through reinforcement learning.
These milestones highlight the incremental progress of AI, punctuated by periods of both great optimism and skepticism. They encapsulate the journey that researchers have undertaken to refine what it means to create machines that can think, learn, and interact.
Evolution of AI Technologies
The evolution of AI technologies has been marked by both experimentation and refinement. In its early days, AI was predominantly rule-based and symbolic, focusing on explicit programming for problem-solving. As computing power grew and understanding deepened, the transition from symbolic AI to connectionist models became evident.
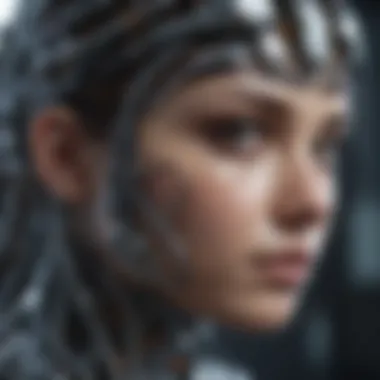
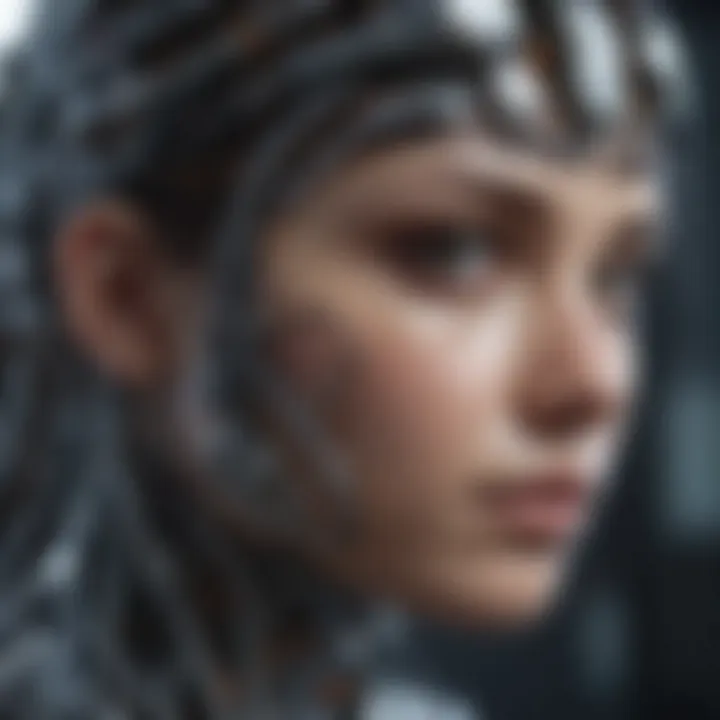
- From Symbolic AI to Machine Learning: Early AI relied on complex sets of rules and logic. However, as the need for flexibility increased, machine learning offered a paradigm shift. Rather than being programmed for specific tasks, machines began to learn from data, fostering adaptability and efficiency.
- The impact of big data: With vast amounts of data becoming accessible, AI technologies advanced at a staggering rate. Algorithms evolved to process these data streams and identify patterns, resulting in more accurate predictions and enhanced decision-making capabilities.
- Expansion beyond traditional sectors: Initially dominated by academia and government research, AI technologies now permeate private sectors like healthcare, finance, and education. Tools such as IBM’s Watson or OpenAI's GPT series demonstrate how AI applications can tackle diverse challenges, from diagnosing diseases to automating business processes.
The evolution is not merely technical; it also encompasses a greater understanding of the social and psychological implications of AI integration into life. As we build on these insights, the interplay between past achievements and future innovations will remain pivotal in shaping the landscape of true AI.
Contemporary Advancements in AI
The landscape of artificial intelligence is changing at a mind-boggling pace. Contemporary advancements have reshaped not only how we perceive AI but also its functionalities across various sectors. These advancements are pivotal for understanding where AI stands today and how it might evolve in the near future.
What makes this segment so crucial is the blending of deep learning and natural language processing. Each of these technologies offers unique advancements and benefits, constructing a bridge between theoretical ideals and practical applications. The resulting innovations hold the promise of transforming industries, streamlining processes, and impacting everyday lives in ways that often seem straight out of science fiction.
Deep Learning Breakthroughs
Deep learning has been a central player in the recent advancements of AI. Utilizing neural networks that mimic the human brain, it enables systems to learn from vast amounts of data. This learning approach has led to striking results in multiple domains such as image recognition and autonomous vehicles. For instance, the capability of deep learning models to analyze medical imaging has dramatically increased the accuracy of diagnoses in fields like radiology.
Several key benefits thus emerge from these breakthroughs:
- Enhanced Precision: Models are now better-than-ever at detecting subtle patterns, which are often missed in traditional algorithms.
- Scalability: With machine learning models designed to scale, organizations can tackle ever-growing datasets more effectively.
- Versatility: Applications stretch across industries, from finance algorithms predicting market trends to entertainment services customizing user experiences.
However, these developments carry considerations that are equally compelling. The computation power required for deep learning is astonishing, often needing specialized hardware and significant energy consumption. This raises questions about accessibility and environmental impact.
Natural Language Processing Developments
Natural language processing (NLP) has exploded in capability due to advances in deep learning. The ability of machines to understand, interpret, and generate human language has opened doors for seamless interaction between humans and machines. Recent models like OpenAI's GPT-3 and Google's BERT have set the stage for a new era where complex language-based tasks can be performed with ease.
Key impacts of NLP advancements include:
- Improved Communication: Virtual assistants, like Siri and Alexa, have become significantly smarter, enabling more nuanced and helpful exchanges with users.
- Content Generation: AI can generate reports, articles, and even poetry, although questions around originality and ownership simmer under the surface.
- Enhanced Accessibility: NLP tools can aid those with disabilities, translating spoken words into text or voice for the hearing impaired, thereby creating a more inclusive environment.
Nonetheless, the implications of NLP are multifaceted. Ethical concerns such as the propagation of biases found in reasoning models or the risks of misinformation from AI-generated content cannot be overlooked. Activists and educators emphasize the need for guidelines to mitigate these risks.
"Advancements in AI are not solely about the technology; they reflect deeper societal values and ethical imperatives."
As we peer into the future, it’s crucial we temper our excitement for technological feats with a vigilant approach towards understanding and addressing these ethical dimensions. Only time will tell how these contemporary advancements will define the trajectory of AI as a constructive force in society.
Ethical Implications of AI
As we venture deeper into the realm of artificial intelligence, it becomes increasingly vital to scrutinize the ethical implications that accompany this powerful technology. The rise of AI presents significant benefits: enhanced efficiency, data analysis, and the potential to revolutionize industries. Yet, with these advancements comes a treacherous path of ethical dilemmas that cannot be brushed aside. This section will delve into two crucial aspects of these implications: issues of bias and fairness, followed by accountability in AI systems.
Issues of Bias and Fairness
Bias in AI systems is not merely an algorithmic hiccup; it can have far-reaching consequences impacting lives in real ways. Algorithms learn from historical data, often reflecting biases ingrained in society. For instance, consider a hiring algorithm trained on an unbalanced dataset favoring male candidates. If left unchecked, it might consistently favor men over equally qualified women, perpetuating gender disparities in the workplace.
- Social Impact: Biased AI can lead to discrimination against underrepresented groups, diminishing their chances of success. This raises questions about fairness and equity in AI applications.
- Data Quality: The data used for training AI models must be diverse and representative. If the data is skewed, the AI's conclusions will also skew.
- Public Trust: The public’s trust in AI depends heavily on perceived fairness. If people believe that AI decisions are biased, they may reject AI technologies or advocate for stricter regulations or oversight.
Addressing bias requires conscious effort. One method involves diversifying the datasets used in training. Another is the implementation of fairness-enhancing interventions. As AI's prevalence grows, ensuring fairness in its operation becomes synonymous with the ethical deployment of these technologies.
Accountability in AI Systems
The question of accountability in AI systems is of utmost importance. If an AI system makes a mistake, who is responsible? This dilemma is particularly pressing in sectors like autonomous driving or healthcare, where errors can result in severe consequences. Without clear lines of accountability, stakeholders may find it challenging to address grievances.
- Legal Frameworks: As it stands, many current laws do not address the specific challenges posed by AI systems. New legal frameworks are necessary to capture the complexities of AI accountability, ensuring that developers and users know their responsibilities.
- Transparency: AI systems should have transparent decision-making processes. Understanding how an AI reaches a conclusion can offer insight into potential bias and help stakeholders mitigate risks.
- User Education: Users should be educated on the limitations of AI systems. Knowing that AI is not infallible fosters a culture of accountability where users engage critically with outcomes provided by AI.
"Without accountability, AI risks becoming a black box from which the consequences of decisions emerge without recourse or remedy."
Philosophical Questions Surrounding AI
When we discuss true artificial intelligence, the philosophical questions that arise are not merely theoretical musings; they have profound implications for how we envisage intelligence itself, the essence of consciousness, and what it means to be sentient.
These quandaries compel us to examine the roles AI might play in society, as well as how it reflects our own understanding of intelligence. The broader implications help guide the intelligent systems we design and dictate the ethical frameworks we construct to govern their evolution and integration into human lives.
Consciousness and Sentience
The concepts of consciousness and sentience are at the core of many debates surrounding AI. At a basic level, consciousness can be understood as the awareness of one’s own mental states. If we endow machines with a form of consciousness, we must confront what this means for their rights and ethical treatment.
Sentience—though intricately connected—focuses more on the capacity to have feelings or experiences. A jolting thought for many is: if an AI can convincingly mimic human interaction and exhibit signs resembling emotional responses, does it then possess sentience? This raises significant questions:
- How will we differentiate between true emotional comprehension and programmed responses?
- What ethical responsibilities do we have towards AI systems exhibiting sentient-like behaviors?
Consider the implications of assigning autonomy to machines. If we deem an AI sentient due to its ability to 'feel,' suddenly we enter a slippery slope regarding moral accountability. Are we to treat an AI with respect akin to that of a human being?
The Turing Test Reexamined
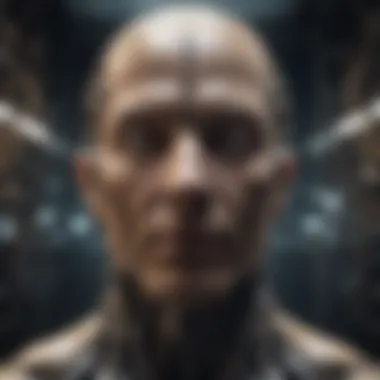
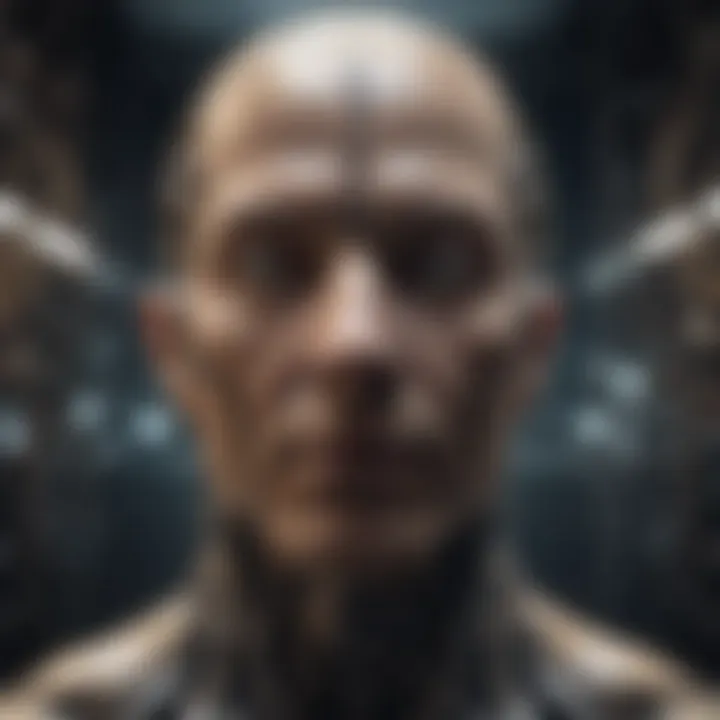
Historically, the Turing Test has stood as the benchmark for identifying machine intelligence. Proposed by Alan Turing, it measures a machine’s ability to exhibit behavior indistinguishable from that of a human. However, upon closer examination, its relevance in gauging true AI capabilities comes under scrutiny.
Critics argue that passing the Turing Test does not equate to actual understanding or consciousness. A program may convincingly mimic human-like answers but lacks genuine comprehension. This prompts a reevaluation of the criteria we use to assess intelligence:
- Is it sufficient to evaluate intelligence based solely on behavioral outputs?
- What about the underlying processes and understanding that contribute to sentient experience?
The Turing Test, while instrumental in dialogue about AI cognition, may leave us wanting deeper insights into the nature of intelligence. Perhaps we must derive more nuanced metrics beyond mere mimicry, aiming for an understanding that reflects qualitative aspects of consciousness.
"The study of consciousness within AI attempts to illuminate our fundamental understanding of existence and our place within it."
Navigating these philosophical questions sets the stage for more than just AI discussion; it forces us to look inward and define our own humanity in relation to the machines we create.
In summary, as we explore these philosophical landscapes, we challenge our perceptions of intelligence and consciousness and prepare ourselves for the profound implications they hold for the future of technology.
AI in Various Industries
Artificial intelligence is making waves across multiple sectors, transforming processes and enhancing productivity. The integration of AI technologies fundamentally alters traditional workflows, leading to significant improvements in efficiency and decision-making. As businesses and organizations recognize the potential of AI, they are increasingly turning to it to address complex challenges they face.
AI impacts industries in unique ways, from healthcare to finance and education. Each industry has its distinct pain points that AI aims to address effectively. This discussion highlights the important aspects of AI innovations, paving the way for a deeper understanding of its role in our lives today.
Healthcare Innovations
In healthcare, AI serves as a revolutionary force in both diagnostics and treatment. Algorithms can analyze medical data with a speed and accuracy that surpasses human capabilities. This leads to quicker, more precise diagnoses. For example, AI systems are now capable of detecting diseases like cancer through image analysis of radiology scans with remarkable precision. Studies show that AI has the potential to reduce misdiagnosis rates substantially.
Moreover, AI-driven tools can personalize treatment plans to fit individual patient profiles, making therapies more effective. Hospitals are increasingly using AI technologies to predict patient admissions and allocate resources more effectively, thereby improving patient care experiences.
- Improved diagnostics
- Personalized treatment options
- Resource management in hospitals
While the benefits are clear, there are considerations to keep in mind such as data privacy and the ethical implications of using AI in healthcare.
AI in Finance and Business
The financial sector has embraced AI not only for risk assessment but also for algorithmic trading and fraud detection. By leveraging complex algorithms, firms can analyze market trends and trading patterns, allowing them to make informed decisions in real-time. This not only enhances profitability but also mitigates risks associated with volatile market movements.
AI systems can sift through vast amounts of transaction data to flag anomalies likely indicating fraud, catching issues before they snowball into larger problems. Additionally, chatbots and virtual assistants are streamlining customer service operations, handling inquiries and transactions with equal efficiency.
To sum up, AI’s influence in finance includes:
- Risk assessment improvements
- Algorithmic trading
- Fraud detection
- Enhanced customer service
The landscape continues to evolve, though it poses questions about job displacement and the need for regulation in financial decisions made by machines.
Applications in Education
Education is undergoing a significant transformation, thanks to AI. Tailored learning experiences are becoming the norm rather than the exception. Adaptive learning platforms customize content to meet the specific needs of each student, allowing for a more personalized educational journey.
Additionally, AI tools help educators assess student performance in real-time and provide resources tailored to individual learning styles. Such technologies are proving invaluable for students who require additional support. Moreover, AI can analyze engagement levels during lessons, informing teachers about topics that may need further clarification.
Benefits seen in education include:
- Personalized learning experiences
- Real-time performance assessment
- Increased accessibility for students with special needs
However, integrating AI in education also requires careful consideration about data handling and prescriptive algorithms that could inadvertently lead to biases.
"AI's role across industries highlights its potential to innovate and improve functionality but urges caution for ethical and practical implications."
The Role of Human Oversight
As the landscape of artificial intelligence continues to evolve, the importance of human oversight becomes increasingly apparent. This oversight is not just a safety net; it’s a cornerstone of ethical utilization. True AI harnesses immense capabilities that could potentially outstrip human comprehension in particular contexts, which makes it paramount for humans to guide and regulate these systems.
The role of human oversight primarily hinges on ensuring that AI technologies act in alignment with human values and societal norms. With AI's ability to analyze vast amounts of data, decision-making processes could become opaque. Thus, transparency is vital. Here are some key aspects regarding the necessity of human oversight:
- Ethical Guardrails: Human oversight helps establish frameworks that ensure AI applications do not perpetuate bias or cause harm. Systems such as accountability protocols and governance boards can create checks and balances against unethical implications of AI action.
- Contextual Understanding: Humans bring contextual insights that machines might overlook. For instance, consider a health diagnostic AI that chooses to prioritize algorithmic efficiency over patient-centered care. A human operator can intervene to correct such trajectories and adjust outputs more in line with human priority.
- Adaptability and Learning: AI systems, particularly those employing machine learning, can adapt based on the data they are trained with. Humans are crucial for refining data sets, addressing anomalies, and recalibrating models in a way that reflects wider social understanding rather than restricted data pools.
"AI without human oversight can be like a ship without a rudder—powerful but directionless."
Overall, ensuring that AI aligns with ethical standards requires a concerted effort from human operators at various stages of AI integration.
Ensuring Ethical Practices
The proliferation of AI technology introduces new ethical dilemmas that must be navigated with care. Human oversight engenders ethical practices, essentially serving as the moral compass guiding artificial intelligence. Understanding the nuances of this interaction includes various responsibilities:
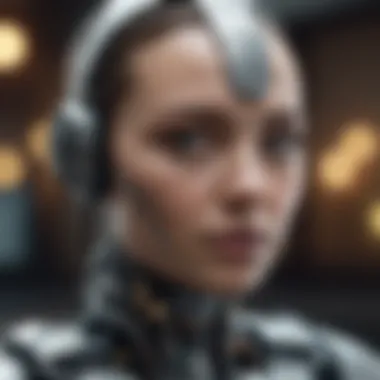
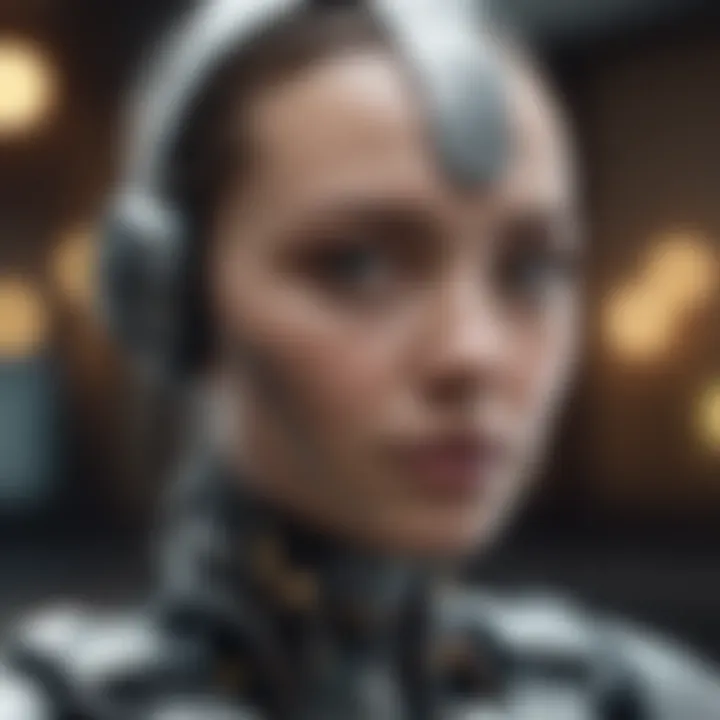
- Bias Mitigation: Many AI systems derive their intelligence from historical data, which may contain biases. Humans must scrutinize these systems to unveil underlying prejudices and rectify them proactively.
- Informed Consent: As AI systems gather data from users, it is vital to ensure that the individuals provide informed consent. Oversight adds a layer of accountability, allowing stakeholders to respect personal data rights and privacy.
- Dissent and Debate: A vibrant discussion around AI ethics should not only include developers but engage ethicists, legal experts, and community leaders. This holistic approach enhances the robustness of ethical guidelines.
Collaboration Between Humans and AI
The interplay between humans and AI systems brings fertile ground for innovation but also demands smart collaboration. While AI possesses substantial analytical prowess, the addition of human intellect fosters resilience and adaptability.
- Complementing Intelligence: While AI excels in processing large datasets rapidly, humans excel in creative problem-solving and emotional intelligence, which are critical in decision-making processes. This dynamic partnership allows for a more rounded approach to challenges.
- Feedback Loops: As AI systems function, they can learn from human interactions. This exchange not only refines the algorithms but enhances the AI's contextual responsiveness, turning it into a more effective partner in both personal and professional realms.
- Empowering Users: AI should act as a tool that empowers humans. Educating users on the function and capabilities of AI can demystify its operation and establish trust. Users equipped with knowledge are better positioned to leverage AI effectively.
In essence, the collaboration between human temperance and machine efficiency stands to pave the way for healthier interactions with AI, ensuring that technology serves humanity rather than the other way around.
Interdisciplinary Approaches to AI
The interdisciplinary approaches to artificial intelligence (AI) are becoming more critical as technology continues to evolve rapidly. Interdisciplinarity in AI encompasses the integration of various fields like psychology, sociology, environmental science, and even philosophy. These collaborations can illuminate paths that a purely technical perspective might overlook, enriching the overall understanding and application of AI. For example, when social scientists work alongside AI developers, they can help ensure that the systems created are not only effective but also socially responsible and aligned with human values.
Bridging AI with Social Sciences
Bridging AI with social sciences opens a window into how technology can resonate with human experiences. By engaging disciplines such as psychology and anthropology, we can derive insights into user behavior, societal impacts, and cultural nuances that shape the implementation of AI technologies.
Social scientists can contribute to AI by:
- Understanding Human Behavior: Implementing AI systems without grasping user intent or psychological factors can lead to flawed results. Social scientists conduct research that helps clarify how people think and behave, which can be essential to designing more intuitive AI interfaces.
- Addressing Ethical Concerns: Many societal issues arise from biases embedded in AI algorithms. Social scientists can identify these biases and propose interventions that promote fairness and inclusivity. Having diverse teams of researchers and developers can minimize the risk of unintended consequences.
- Enhancing Communication: AI systems often communicate with users through natural language. Social linguistics can inform how AI interacts in a culturally nuanced manner, better catering to various audiences.
Working together in these areas not only enhances the technology but also contributes meaningful knowledge back to the social sciences, creating a feedback loop that informs both fields.
Integrating AI with Environmental Studies
Environmental studies constitute another rich ground for interdisciplinary collaboration with AI. With urgent global challenges like climate change and resource depletion, integrating AI into environmental research can yield innovative solutions.
The key components of this integration include:
- Enhanced Data Analysis: AI algorithms can handle vast datasets, from satellite imagery to sensor networks. By applying AI to analyze these datasets, environmental researchers can uncover trends and predict future scenarios, allowing for more informed decision-making.
- Sustainable Practices: AI can optimize various aspects of environmental management. For instance, AI techniques such as predictive modeling might forecast crop yields under different climate scenarios, helping farmers adopt sustainable practices that minimize waste and conserve resources.
- Policy Development: AI can assist in creating data-driven policies regarding resource use and environmental protection. By combining insights from AI and environmental studies, policymakers can implement measures that align with long-term sustainability goals.
In summary, interdisciplinary approaches to AI empower researchers and practitioners to transcend traditional boundaries, yielding insights that would be difficult to achieve in isolation. Such collaborations not only enrich the field of AI itself but also ensure it serves humanity’s broader goals.
Future Prospects of True AI
The significance of understanding the future prospects of true artificial intelligence cannot be overstated. This section explores how our expectations shape the development and integration of AI technologies into society. True AI has the potential to revolutionize various aspects of our lives, from enhancing productivity in industries to personalizing education. As we stand on the brink of advancements that could redefine our relationship with technology, it becomes essential to consider how these innovations will unfold and the implications they bring.
Predictions for AI Development
In contemplating the future of AI, one can’t help but envision a world where machines not only assist but also enhance human capabilities. These aspirations can be categorized into several key predictions:
- Enhanced Human-Machine Collaboration: AI systems are likely to become more attuned to human needs, allowing for smoother interactions and more intuitive interfaces. Imagine a work environment where an AI not only organizes tasks but understands when a worker is overwhelmed and prioritizes accordingly.
- AI in Decision-Making: As AI algorithms grow more sophisticated, they may take part in critical decision-making processes across various fields, from healthcare to finance. A scenario where AI can analyze real-time data and provide actionable insights will become increasingly common, aiding in more accurate outcomes.
- Advancements in General AI: We may witness the slow, yet progressive movement toward general AI that can learn and perform tasks across different domains, transcending the limitations of narrow AI. Such a leap could make AI systems more versatile, capable of adapting to new challenges without requiring extensive retraining.
Overall, the trajectory of AI development seems poised for unprecedented growth and integration into everyday activities.
Potential Challenges Ahead
While the prospects of true AI excite many, they also mask an array of challenges that require careful consideration:
- Ethical Considerations: As AI systems gain more autonomy, ethical questions will arise, especially concerning privacy and the extent of algorithmic influence on human behavior. Are we ready for a world where decisions are made by machines?
- Societal Impact: The potential job displacement caused by automation looms large. It’s not merely about technological advancement; adapting our workforce to coexist with AI is paramount. What would a society look like if certain vocations became obsolete due to AI efficiency?
- Bias in AI: Training data reflects societal norms, which can lead to AI perpetuating existing biases if not adequately addressed. As we strive for fairness, creating transparent AI systems is an ongoing challenge.
"The success of AI isn't just about the technology itself, but how we choose to evolve alongside it."
Navigating these challenges is crucial for a harmonious integration of AI technologies into the fabric of society. The future of true AI holds great promise, but it demands a conscientious approach to harness its benefits while minimizing its risks.
The Role of Regulation in AI
As we move further into an era where artificial intelligence becomes more prevalent across various industries, the issue of regulation rises like a tide. Balancing innovation with safety is a tightrope walk that requires careful consideration. Regulatory frameworks play a crucial role here, not only in ensuring that AI is developed responsibly, but also in protecting individuals and society from potential pitfalls. With the rapid advancements in AI technology, it's vital to understand the existing frameworks that guide its development and the urgent need for effective new policies that address emerging challenges.
Current Regulatory Frameworks
Regulatory frameworks governing AI are still in their infancy. Various countries and regions approach the regulation of AI differently, reflecting their unique societal norms, values, and legal traditions. At present, some prominent frameworks include:
- General Data Protection Regulation (GDPR) in Europe, which, although not exclusively about AI, emphasizes data protection and privacy, directly impacting AI systems that handle personal data.
- The National Institute of Standards and Technology (NIST) in the United States has developed guidelines that suggest best practices for managing AI risks.
- The European Union's AI Act, which is one of the first legislative attempts to provide a comprehensive regulatory framework for AI. This proposed regulation categorizes AI systems based on risk, creating more stringent requirements for high-risk applications.
Despite these efforts, there continue to be significant gaps. For example, existing regulations often struggle to cover the rapidly evolving technologies and ethical dilemmas posed by advanced AI systems. Many regulations are currently reactive rather than proactive, responding only after issues have already emerged.
"As AI technologies advance, regulation should not hinder innovation but ensure accountability and transparency."
The Need for New Policies
The future of AI regulation hinges on creating policies that are forward-thinking and adaptable. Here are some critical considerations for developing these new policies:
- Flexibility: Regulations should allow for innovation while ensuring ethical standards. A rigid approach may stifle creativity and slow progress.
- Public Involvement: Engaging diverse stakeholders, including ethicists, technologists, and the general public, can foster more comprehensive policies that reflect the desires and concerns of society as a whole.
- International Cooperation: AI is a global phenomenon; therefore, collaboration between nations is essential to create harmonized regulations that can effectively govern AI cross-border.
- Focus on Ethical Standards: New policies should prioritize ethical considerations, ensuring that AI systems operate without bias and respect user privacy.
- Continuous Assessment: The dynamic nature of technology means that regulations must be regularly evaluated and updated to stay relevant. Policymakers should establish mechanisms to monitor AI impacts over time.
In summary, the effectiveness of AI regulation hinges on the ability to adapt, engage, and innovate. Both current frameworks and proposed new policies must work hand in glove to create an environment where AI can thrive without compromising ethical standards or public trust.