Exploring Careers in Computational Science

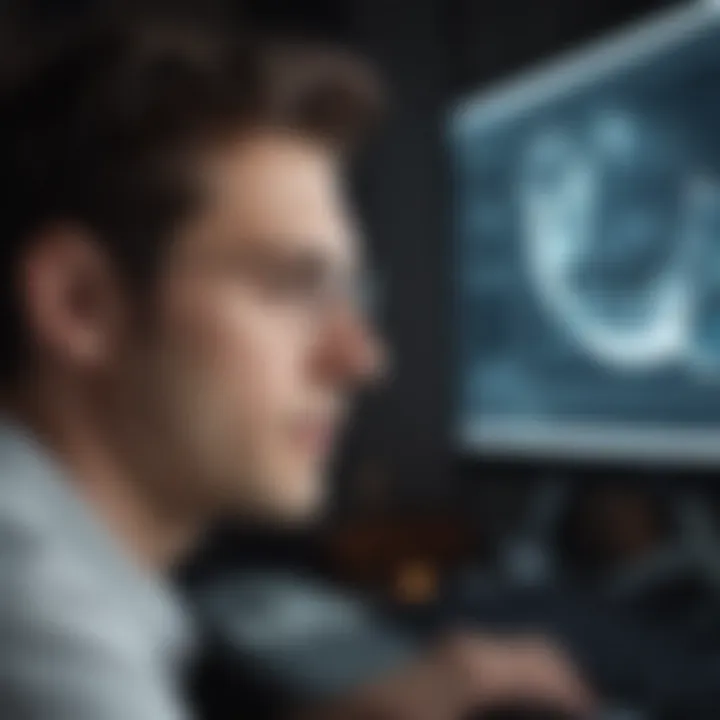
Intro
Computational science has carved a niche in the modern research landscape, fusing traditional scientific principles with massive computational power. This discipline has opened doors to new ways of problem-solving and innovation, deeply influencing various fields like physics, biology, engineering, and social sciences. As the demand for data-driven decision-making surges, the importance of computational scientists becomes increasingly evident.
In a world where every bit of data can yield insights or catalyze solutions, understanding the career prospects within this domain is vital for students, educators, and professionals alike. Exploring careers in this field not only sheds light on the roles available but also emphasizes the unique blend of skills required to thrive. While some may envision computational scientists simply as programmers, the reality is far richer and more complex.
By diving into the multifaceted landscape of computational science careers, we can navigate through essential insights regarding the paths one can take, the tools and methodologies employed, as well as the challenges one might encounter along the way. The journey starts now ā letās unpack what lies ahead!
Research Overview
Summary of Key Findings
The realm of computational science is expansive, encompassing a variety of roles that require a diverse set of skills. Key findings suggest that:
- Computational modelers play a crucial role in hypothesis testing and scenario analysis.
- Software developers in research settings must be well-versed in both programming and scientific domains to effectively bridge disciplines.
- Data analysts are increasingly sought after due to their ability to derive meaningful patterns from massive datasets, which could steer future research directions.
The skills in demand often include programming languages such as Python and R but extend to specialized knowledge in statistics, modeling, and even domain-specific expertise. This hybrid skill set equips professionals to tackle real-world problems efficiently and innovatively.
Background and Context
As the digital age continues to unfold, computational science has emerged not merely as a complementary approach but as a cornerstone of scientific inquiry. The transformation has been driven by technological advancements that have vastly increased our ability to collect, process, and analyze data. Industry giants and academic institutions alike now recognize the value that computational techniques bring to their operations.
The field also reflects the complexities of modern scientific challenges. Issues like climate change, disease modeling, and engineering innovations necessitate collaborative interdisciplinary efforts. Thus, those embarking on careers in computational science stand at the intersection of technology and research, with the potential to significantly impact society.
"The future belongs to those who see possibilities before they become obvious."
Methodology
Experimental Design
To explore the varied careers in computational science, a mixed-method approach combining qualitative and quantitative data was adopted. Interviews with professionals across sectors provided firsthand insights, while surveys highlighted prevailing trends and required competencies across different roles. The focus was on understanding career trajectories, challenges faced, and the daily realities of working in this dynamic field.
Data Collection Techniques
The data collection incorporated several techniques:
- Interviews: Informal discussions with practitioners offered rich narratives about career paths.
- Surveys: Structured questionnaires targeted recent graduates and seasoned professionals, generating quantitative data on skills and job satisfaction.
- Literature Review: Comprehensive analysis of existing reports and studies around computational science careers enriched understanding and provided context.
Through this methodology, a thorough portrayal of careers in computational science emerges, guiding aspiring individuals towards informed career decisions.
Prologue to Computational Science
Computational science is a pivotal field that sits at the crossroads of computer science, mathematics, and the scientific disciplines. Understanding its significance is crucial as the world increasingly leans on computational methods to tackle myriad challenges across various domainsāfrom predicting climate change to designing new drugs. This article aims to peel back the layers of this complex field, providing a clear pathway for those contemplating a career in computational science.
Defining Computational Science
Computational science can be understood as the study and application of mathematical models and computational techniques to solve real-world problems in various scientific domains. Itās not just about coding or running simulations; it's fundamentally about understanding the behavior of complex systems and leveraging that understanding to forecast outcomes or optimize processes.
In essence, computational science transforms theoretical equations into practically usable tools. For instance, consider climate modeling. Instead of just penning down equations on paper, computational scientists create sophisticated simulations that mimic the Earthās atmospheric conditions. These simulations allow researchers to visualize potential future scenarios based on current trends and data, ultimately aiding in decision-making regarding environmental policies.
Historical Development
The roots of computational science stretch back to the early days of computing itself. Initially, the relationship between computers and scientific inquiry was rather simplistic, often limited to numerical calculations. The advent of the electronic computer in the mid-20th century was a game changer.
During the 1950s and '60s, advancements in both hardware and software started to forge a new path. A key moment in this historical journey was the development of numerical weather prediction models, which demonstrated the immense potential of computers in solving complex equations that govern natural phenomena.
As technology burgeoned, computational science evolved to include not just physics and weather forecasting but biology, chemistry, and social sciences. Innovations such as high-performance computing in the 1990s elevated the discipline further, allowing researchers to simulate intricate systems with a level of detail that was previously unimaginable.
This historical context highlights how computational science has emerged as a crucial component of modern research. Today, it is not just about executing algorithms; itās about reshaping our understanding of the universe through simulations and predictive modeling. This evolution not only marks the maturation of a discipline but also reflects the ever-growing demand for computational approaches across a multitude of sectors.
Types of Computational Scientist Jobs
The realm of computational science encompasses a broad spectrum of career paths. Each of these paths offers unique benefits and challenges, contributing significantly to advancements across various fields. Understanding the types of jobs available can help aspiring computational scientists pinpoint where their skills and interests may align best. By recognizing the opportunities in academia, industry, and the government or non-profit sector, individuals can strategically position themselves for rewarding careers.
Academia
In the academic world, computational scientists often push the boundaries of knowledge, utilizing advanced models and simulations to conduct their research. Their roles typically involve teaching, publishing papers, and securing research funding. Pursuing a career in academia can be gratifying, not only because of the intellectual stimulation it provides but also due to the opportunity to mentor future generations of scientists.
Academics often work at universities, where they might lead research teams in fields such as astrophysics or genetics. They may also create new computational methods and tools that can be used for various scientific problems.
The advantages of an academic career include:
- Intellectual freedom: Academics can explore topics they are passionate about, often leading to groundbreaking discoveries.
- Collaborative environment: Working with other researchers can provide invaluable insights and foster creativity.
However, itās worth noting that securing tenure and funding can be fiercely competitive, making this path a double-edged sword for some.
Industry Applications
When it comes to the private sector, computational scientists can find themselves in demand across a variety of industries such as technology, finance, and healthcare. Here, their expertise is often directed towards solving practical problems that can have immediate impacts. For instance, in the tech industry, computational scientists might develop algorithms used in machine learning applications or optimize software performance for better user experiences.
Key roles in industry may involve:
- Data analysis: Interpreting complex datasets to guide business decision-making.
- Product development: Collaborating with engineering teams to enhance existing products or develop new ones based on computational principles.
The industry-focused career provides several benefits, including:
- Higher earning potential: Often, industry positions come with more competitive salaries compared to academia.
- Direct impact: The work done can contribute to products that consumers use daily, making it feel rewarding.
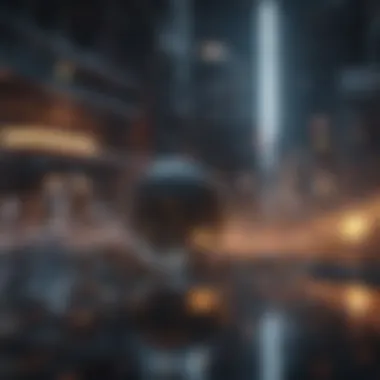
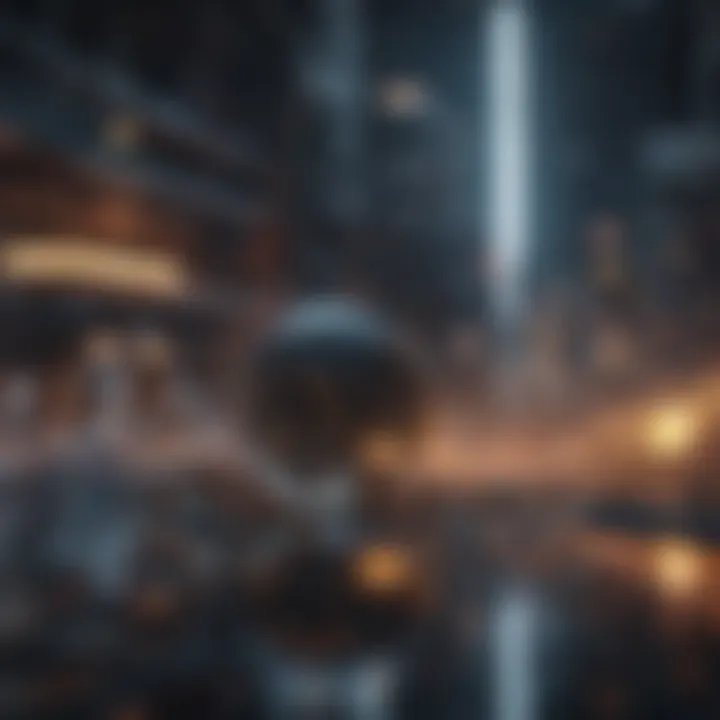
However, the pace in industry can be rapid, and scientists may find themselves working on short-term projects that leave little room for long-term exploration.
Government and Non-Profit Sector
Lastly, the government and non-profit sectors employ computational scientists to tackle societal challenges, ranging from environmental issues to public health crises. Roles here may include research positions at national laboratories, working on simulations for climate modeling, or developing computational tools for health data analysis.
Some key responsibilities in this sector are:
- Policy development: Informing public policy through data-driven recommendations based on computational analysis.
- Public outreach: Educating communities about scientific findings and how they affect everyday life.
Working in government or non-profit organizations often comes with its unique rewards:
- Contributing to the public good: Many find fulfillment in projects that aim to improve society.
- Stability: These jobs may offer a more secure work environment compared to the volatile private sector.
Nevertheless, the funding in these sectors can be limited, and resources may not be as plentiful as in industry.
"Computational science is like a compass that navigates us through the complex waters of modern research, no matter where we choose to dock our ships."
Key Skills Required for Computational Scientists
In the fast-evolving field of computational science, certain skills serve as the bedrock for success. These skills not only enhance an individual's problem-solving abilities but also ensure efficacy in research and application. Having a robust skill set in programming, mathematics, and data analysis is pivotal. This section dives into the specific skills required, highlighting their relevance and utility in various contexts within computational science.
Programming Languages
Python
Python stands out as a programming language that has garnered much attention and acclaim in the field of computational science. Its simplicity and readability have made it a preferred option for both beginners and seasoned professionals. One key aspect of Python is its vast array of libraries and frameworks, such as NumPy and SciPy, which facilitate complex mathematical computations and data manipulation effortlessly. This makes it not just a beneficial choice but almost essential for anyone looking to dabble in data science or numerical modeling.
An intriguing feature of Python is its community support. Users can easily find libraries tailored for various niches, from web scraping to machine learning. However, while Python shines in versatility and ease, it may not be the best choice for performance-critical applications. In high-stakes environments, its interpreted nature can lead to slower execution compared to compiled languages. Nonetheless, for the majority of computational science applications, the benefits of Python far outweigh its drawbacks.
++
C++ is another heavyweight in the world of programming languages, renowned for its performance and control over system resources. A defining characteristic of C++ is its ability to handle low-level programming, making it invaluable for computational heavy lifting when optimized performance is necessary. This translates well into fields like simulation modeling and systems programming.
The unique feature of C++ lies in its object-oriented nature, allowing for the creation of complex software solutions. This can lead to more maintainable and reusable code in large projects. However, the steep learning curve can pose challenges for newcomers, particularly those transitioning from more accessible languages like Python. Despite this, for those willing to invest the time, C++ can be a key asset in the computational scientist's toolkit.
R
When it comes to statistical analysis and data visualization, R is often the first choice among computational scientists. It offers a comprehensive ecosystem for statistical computing that is especially beneficial for academic research. One key feature of R is its extensive collection of packages, catering to almost any statistical method imaginable, which is a boon for researchers looking to analyze data rigorously.
R excels particularly in the realm of data visualization, with packages like ggplot2 that allow for highly customizable graphs and plots. However, one of its limitations is that its performance can lag when handling very large datasets compared to other languages like Python or C++. Still, for specific statistical tasks, R remains an indispensable tool.
MATLAB
MATLAB is widely recognized for its capability in matrix calculations and algorithm development, which makes it immensely popular in engineering and scientific communities. One of its standout features is its user-friendly interface and numerous built-in functions that simplify complex mathematical computations. This is particularly advantageous for researchers and engineers who may not prioritize programming skills as much as scientific accuracy.
Importantly, MATLAB's strong emphasis on numerical analysis means it can be a great choice for prototyping and testing algorithms rapidly. However, its commercial licensing can be a barrier compared to the open-source alternatives. For institutions and companies that already have access, the convenience of MATLAB can be a significant asset in computational endeavors.
Mathematical and Statistical Proficiency
Mathematics forms the backbone of computational science, acting as the language through which scientists model complex problems. Essential aspects include numerical methods, statistical theory, and linear algebra. A solid foundation in these areas empowers computational scientists to create robust models, analyze data, and interpret their findings effectively. Understanding statistical principles helps in drawing valid conclusions from data analyses, reducing the risk of misleading results that can stem from poor methodological choices. Moreover, mathematical models often provide the structure needed to guide programming efforts, ensuring that computations align with theoretical expectations.
Data Analysis Techniques
Data analysis techniques are crucial for deriving meaningful insights from raw data. Mastery of these techniques enables computational scientists to identify patterns, make predictions, and inform decision-making. Familiarity with methods such as regression analysis, time-series analysis, and clustering algorithms can be pivotal in various applications, from analyzing biological data to optimizing engineering processes.
One noteworthy method includes machine learning, which involves training algorithms on datasets to make predictions or classifications. The versatility of these techniques opens doors to exciting opportunities in various disciplines, including finance, healthcare, and climate science. However, computational scientists must remain cautious and ensure that they choose the right technique for the specific problem at hand, as applying a one-size-fits-all approach can lead to flawed conclusions.
Educational Pathways
Navigating a career in computational science involves more than just a passion for technology and a thirst for knowledge; it heavily relies on structured educational pathways. This segment will dive into the cornerstone of careers in this field, showcasing the various educational routes that aspiring computational scientists can take. From the foundational underpinnings of undergraduate programs to advanced studies and ongoing professional development, each path plays a vital role in shaping a well-rounded professional equipped to tackle the complexities of computational science.
Undergraduate Programs
Undergraduate programs set the stage for foundational knowledge in computational science. A bachelor's degree, whether in computer science, mathematics, physics, or a related discipline, lays the groundwork. Consider this: a degree in computer science can provide insights into algorithms and coding, which is fundamental for computational modeling. Meanwhile, a degree in mathematics could offer deeper understanding of statistical analysis, critical for data interpretation.
Take a closer look at some degree programs that can steer students toward a successful career:
- Computer Science: Focuses on programming, systems design, and algorithm development.
- Mathematics: Delves into theories and practical applications of mathematical principles.
- Physics: Merges fundamental laws of nature with computational tools for simulations.
- Engineering: Emphasizes practical skills and problem-solving using computational techniques.
each of these disciplines brings something unique to the table, fostering a skill set tailored for computational applications.
Graduate Studies
Earning a master's or doctoral degree can often be the game changer for those serious about making an impact in computational science. Graduate studies enable specialization that undergraduate programs generally do not offer. For instance, a master's in computational biology centers on practical applications of computational techniques in life sciences, while a PhD program allows for intensive research in niche areas like machine learning or fluid dynamics.
Prospective students should ponder over their career aspirations when considering these advanced degrees. A focused program can lead to industry-ready professionals or researchers equipped to contribute significantly to academia or innovation sectors.
Here's what to consider:
- Research Opportunities: Engaging in research during graduate school can provide invaluable experience.
- Interdisciplinary Approach: Many programs encourage collaboration across fields, enriching the learning experience.
- Networking Prospects: Graduate studies often facilitate connections with industry and academia, essential for job hunting later on.
Professional Development and Certifications
As technology evolves, so does the landscape of computational science. Ongoing professional development through certifications and workshops can help scientists stay ahead of the curve. Certificates in areas like data science or cloud computing can supplement a traditional degree and demonstrate to employers a commitment to progressing in the field.
Furthermore, professional development serves as a practical way to explore new subjects without the overhead of a degree program. Here are some recommended avenues:
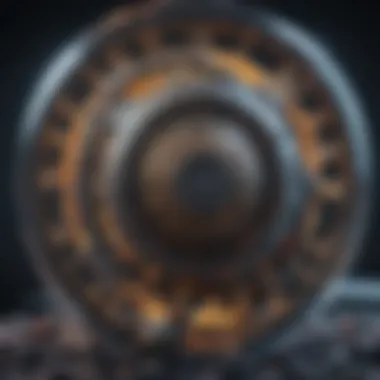
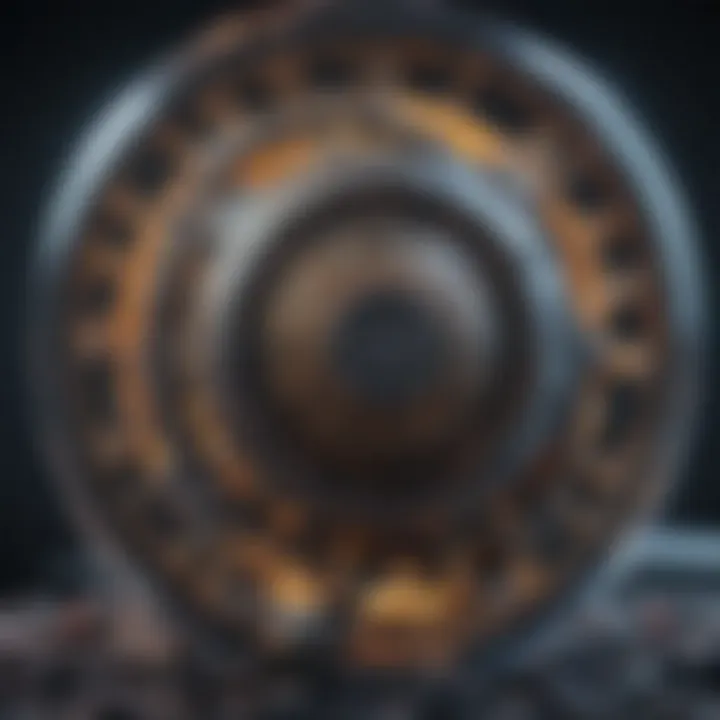
- Online Platforms: Websites such as Coursera and edX offer numerous courses tailored for professional growth.
- Industry Certifications: Organizations like Microsoft and AWS provide certifications that are highly regarded in the tech industry, ensuring competencies align with current market demands.
- Workshops and Bootcamps: Engaging in workshops facilitates hands-on experience with cutting-edge tools and software.
"Educational pathways are not merely means to earn a degree; they are integral to building a solid foundation and growing within the field."
In summary, educational pathways in computational science vary greatly, each offering distinct opportunities and benefits. By making informed choices regarding undergraduate programs, pursuing advanced degrees, and embracing lifelong learning through professional development, individuals can equip themselves with the tools necessary to thrive in this dynamic field.
The Role of Computational Science in Different Disciplines
Computational science plays a pivotal role across numerous fields, often acting as the bridge that connects theoretical concepts with real-world applications. Itās not just about crunching numbers; itās about understanding complex systems and finding solutions to intricate problems that could previously only be theorized. As various industries lean increasingly into data-driven decision-making, the relevance of computational science becomes more pronounced.
In disciplines like physics, biology, and chemistry, computational methods enable researchers and practitioners to model scenarios, predict outcomes, and simulate experiments that may be impractical or even impossible to conduct in real life. This capability not only enhances research efficiency but also fosters innovation and drives advancements in technology, health, and environmental sustainability.
Physics-Based Applications
When we talk about physics, itās essential to highlight that many phenomena are too vast or minute for hands-on experimentation. In fields such as astrophysics, computational scientists simulate celestial events or gravitational interactions, allowing for deeper insights into the mechanics of the universe. For instance, the simulation of black hole dynamics or cosmic microwave background radiation helps physicists make decisions that are supported by data rather than mere hypotheses.
Furthermore, these simulations play a crucial role in materials science. By predicting how materials will react under different conditions, scientists save time and resources in the development of new substances. This intersects closely with industries like aerospace, where understanding material strength under stress can lead to safer and more efficient spacecraft.
Biological Research
Bioinformatics is a thrilling field that heavily relies on computational science. With the human genome project as a landmark achievement, researchers now utilize algorithms to analyze vast datasets from DNA sequencing. This has paved the way for personalized medicine, allowing doctors to tailor treatments based on an individual's genetic profile. The implications here are massive, affecting everything from drug design to the understanding of evolutionary biology.
Additionally, computational models are employed in epidemiology to track disease outbreaks. By simulating the spread of infectious diseases, public health officials can make informed decisions on resource allocation and containment strategies. In essence, computational science provides the tools needed to tackle health crises from data perspective, ensuring a better-prepared response system.
Chemical Engineering and Simulations
In chemical engineering, computational science is indispensable. From simulating chemical reactions to optimizing industrial processes, computational tools help predict outcomes, reduce waste, and maximize efficiency. For example, computational fluid dynamics (CFD) is used to streamline processes in petroleum refining or in the design of reactors. By predicting how substances will behave under various conditions, these simulations not only enhance productivity but also ensure that safety is never compromised.
Moreover, environmental chemistry is benefitting from these technologies too. Simulating pollutant behavior in water and air allows for a clearer understanding of its impact and enables the formulation of effective remediation strategies.
"Computational science not only amplifies our understanding of complex systems but also expedites the discovery of groundbreaking solutions across disciplines."
In summary, the role of computational science in diverse disciplines cannot be overstated. It stands as a crucial ally in scientific research, propelling the boundaries of what we know, improving methodologies, and directly impacting society's challenges. Whether it is through enhancing our understanding of the universe or driving innovation in health and engineering, the opportunities rooted in computational science provide a promising horizon for future advancements.
Advancements in Computational Tools and Technology
Advancements in computational tools and technology are reshaping how scientists and professionals in various fields approach problems, analyze data, and communicate results. These innovations serve as a backbone to the exponential growth in computational science, enabling high-precision calculations, complex simulations, and more effective data handling. The increase in computing power helps to address some of the modern scientific challenges while boosting productivity and efficiency across numerous industries.
High-Performance Computing
High-performance computing (HPC) acts as a key player in the advancements within computational science. By utilizing supercomputers and parallel processing techniques, HPC allows computational scientists to solve problems that require extensive calculations, which traditional computers canāt handle efficiently.
- Benefits of High-Performance Computing:
- Speed: Tasks that previously took days or weeks can now be completed in a fraction of the time, amplifying research outputs.
- Complex Simulations: Researchers can create more intricate models that mimic real-world phenomena, improving the predictive power of their studies.
- Handling Big Data: Applications processing immense datasets benefit tremendously from HPC, which aids in extracting meaningful insights without the bottlenecks usual in data processing.
HPC has significant implications in fields like climate modeling, genomics, pharmaceuticals, and engineering simulations, where one needs to weigh tons of data quickly to make informed decisions. It is important to know that even with all its advantages, HPC requires substantial investment in infrastructure, training, and maintenance, which poses challenges for smaller institutions.
Machine Learning and AI Integration
The rise of artificial intelligence (AI) and machine learning (ML) has fundamentally altered computational methods and enhances predictive analytics across various disciplines. Integrating ML into computational science offers transformative possibilities because it enables the development of models that can learn from data autonomously.
- Key Aspects of ML and AI Integration:
- Efficiency in Data Analysis: These technologies speed up the data analysis processes, reducing time spent on manual analysis.
- Pattern Recognition: AI excels at identifying subtle patterns or anomalies in large datasets that may elude traditional statistical methods.
- Adaptive Algorithms: Machine learning algorithms can evolve as more data becomes available, continuously improving predictions and insights over time.
The application of ML in fields like biomedical research, financial modeling, and environmental monitoring showcases its versatility. Yet, computing experts need to remain vigilant regarding the ethical implications and biases that might arise from automated decision-making systems.
Cloud Computing and Accessibility
Cloud computing has become integral in making computational tools and resources more accessible. It provides the necessary scalability, flexibility, and collaboration opportunities that significantly democratize access to sophisticated computing technologies.
- Negatives of Cloud Computing:
- Lower Initial Costs: There is no need to invest heavily in hardware, making it easier for startups and individual researchers to get involved in complex computational tasks.
- Collaboration Opportunities: Shared workspaces in the cloud facilitate joint research efforts, allowing teams across multiple locations to contribute in real-time.
- Resource Availability: Users can tap into vast computing resources on-demand, enabling them to tackle more extensive projects than what their local machines could manage.
However, while cloud computing paves the way for ease of access, it does also come with drawbacks such as data security concerns and potential loss of control over sensitive data.
Challenges Faced by Computational Scientists
The journey of a computational scientist isn't just about crunching numbers and developing algorithms. It's also about navigating a complex landscape filled with challenges that can significantly affect not only their work but also the broader impact of their research. Understanding these hurdles is critical for those aspiring to enter this field, as it allows them to prepare for the realities of their chosen career. The challenges faced can range from managing large volumes of data to ensuring that research findings can be reproduced reliably. Here, we delve into some of the key challenges that computational scientists must address.
Data Management Issues
Data is the lifeblood of computational science. However, the sheer scale and complexity of data can create significant management challenges. In many cases, scientists need to handle datasets that are not only vast but also messy and inconsistent. The issues can include:
- Data storage: Keeping data secure while also making it accessible for analysis.
- Data documentation: Ensuring that datasets are thoroughly annotated so others can understand the context in which they were generated.
- Data integrity: Maintaining accuracy and consistency, particularly when data is collected from multiple sources.
These factors can lead to challenges in collaboration, as scientists work often in teams or across projects. Without a strong foundation in data management, the integrity of research can come into question, potentially undermining the value of findings when others attempt to build upon them.
Reproducibility in Research
Reproducibility is a cornerstone of scientific inquiry. In the world of computational science, this concept takes on an added layer of complexity. It is not just enough for research to be fully explained; the methods also need to be preserved and accessible. Key concerns regarding reproducibility include:
- Methodological transparency: During the development of computational models, itās essential to document every step painstakingly. Failing to do so can lead to discrepancies if others try to replicate the experiments.
- Version control: Keeping track of changes in code and models is crucial. Without this, small modifications can lead to large variations in outcomes, thus affecting reproducibility.
- Standardization: Utilizing standardized protocols and libraries enables better collaboration and tracing of methods used in experiments.
As computational scientists strive to ensure that their work can be independently verified, overcoming the challenges of reproducibility serves to strengthen the credibility of research in their field.
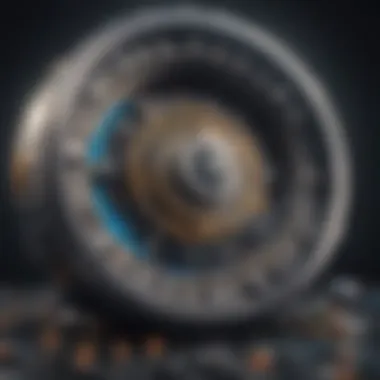
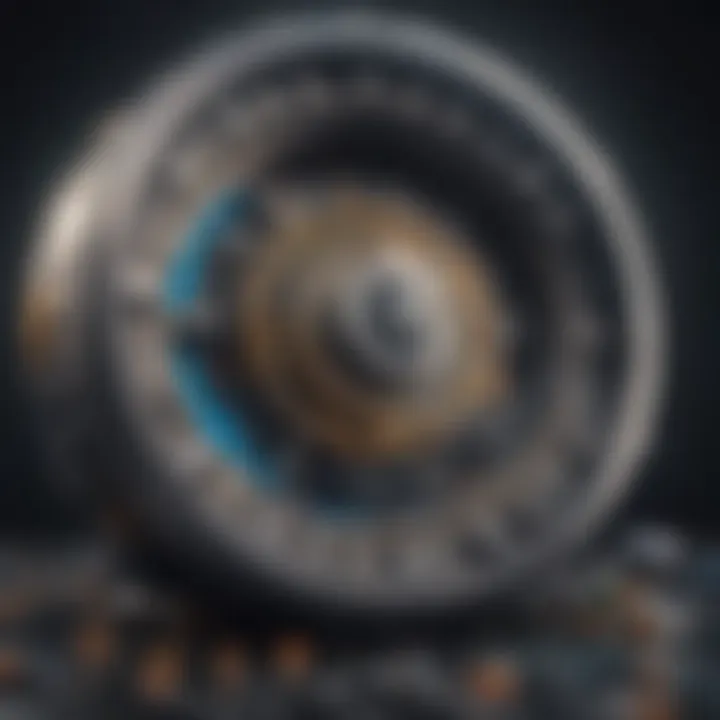
Ethical Considerations
In an era where technology continues to evolve rapidly, ethical considerations in computational science have become paramount. With the power of computation comes a responsibility that scientists must uphold. Several key ethical challenges include:
- Data privacy: Handling sensitive data, especially in fields like healthcare, puts scientists at the forefront of ensuring that personal information is protected.
- Misuse of algorithms: As machine learning and artificial intelligence become more integrated into research, the potential for biased algorithms or misinterpretation of data must be addressed.
- Environmental impact: The carbon footprint of high-performance computing is an emerging issue. Computational scientists must look at ways to reduce their impact as they harness the power of computing resources.
"As we continue to deepen our reliance on computational methods, the ethical implications of our work will only grow. It is crucial to integrate profound ethical considerations into our research ethos."
Navigating the ethical landscape requires ongoing discussion and reflection within the scientific community. Facing these challenges directly is vital to not only advance individual careers but to uphold the integrity of computational science as a whole.
In summary, the field of computational science presents a wealth of opportunities, but also its fair share of obstacles. Understanding these challenges equips academia and industry professionals alike to better meet the demands of this evolving landscape.
Career Outlook for Computational Scientists
The realm of computational science is a vibrant and rapidly evolving field. Understanding the job market and the future prospects for computational scientists is crucial for anyone looking to carve out a career in this area. This section delves into the current job market trends and the future opportunities that await professionals with expertise in computational science. Having a clear picture of these aspects allows aspiring computational scientists to make informed decisions about their educational and career paths.
Current Job Market Trends
Recently, the job market for computational scientists has shown a healthy pulse, thanks in part to an exponential increase in data generation across various sectors. The demand for skilled individuals who can interpret, analyze, and draw conclusions from large data sets has surged. Companies are on the lookout for professionals who can harness the power of computational modeling and simulations to solve complex problems.
Several industries are currently leading the charge in hiring computational scientists:
- Technology companies: Whether it's developing algorithms for artificial intelligence or simulating user interactions in software, tech firms are consistently in need of computational expertise.
- Healthcare: With the rise of personalized medicine, computational scientists play a pivotal role in data analysis and modeling to enhance patient care.
- Environmental science: Thereās a growing interest in using simulations to predict climate change impacts, driving demand in environmental research organizations.
Despite the robust demand, itās not all smooth sailing. Competition is becoming fiercer, particularly for high-profile positions in top tech companies and research institutions. Additionally, many of these roles require not just technical skills but also an understanding of specific domains, making interdisciplinary knowledge invaluable.
Future Opportunities
The horizon for computational scientists is bright, with emerging technologies paving the way for new opportunities. Here are key areas where growth is expected:
- Artificial Intelligence and Machine Learning: As AI continues to integrate into every sector, professionals who can develop and understand algorithms will be at a premium. The ability to analyze data and improve learning models will define success in this space.
- Quantum Computing: As quantum technologies inch closer to mainstream application, the demand for scientists proficient in quantum algorithms will rise. This emerging field promises to revolutionize problem-solving capabilities.
- Big Data Analytics: Organizations are increasingly struggling to make sense of vast amounts of data. Proficiency in big data tools and techniques will lead to significant career advancements, as businesses seek out those who can extract insights from data.
"The landscape of computational science is shifting, demanding adaptability and continual learning from its professionals to stay relevant against a backdrop of rapid technological advancement."
The future also holds challenges tied to the ethical use of data and algorithms, fueling a need for scientists who can navigate these complexities responsibly. Furthermore, soft skills such as communication and collaboration will increasingly set candidates apart, complementing their technical abilities.
The career outlook for computational scientists is one full of potential. Staying attuned to market demands and continuously enhancing skills through professional development will be key for those looking to thrive in this exciting field.
Networking and Professional Growth
In the realm of computational science, building a strong network is as essential as possessing technical skills. The fields of computer science, mathematics, and data analysis interface dynamically with various industries. Therefore, connecting with like-minded individuals and professionals can open doors that remain firmly shut without the right contacts. The significant benefits that come from networking can enhance one's career trajectory in myriad ways.
Networking allows individuals to share insights, industry developments, and collaborate on projects. Itās not just about accumulating contacts; itās about establishing relationships that can bear fruit later onābe it mentorship, job referrals, or collaborative research opportunities. When you engage with a community of professionals, you immerse yourself in the latest trends and innovations, keeping your skills and knowledge updated. Given the rapid transformations in computational science, being in the loop becomes invaluable.
Being active in professional networks can also bolster oneās credibility. Connections you make through associations or conferences often enhance your visibility in the field. A recommendation or reference from a respected figure can tip the scales during competitive hiring processes.
Professional Associations
Professional associations play a crucial role in shaping careers in computational science. Membership in groups such as the Association for Computing Machinery (ACM) or the Society for Industrial and Applied Mathematics (SIAM) provide not just credibility, but a plethora of resources that members can leverage.
Some benefits of joining a professional association include:
- Access to exclusive research and publications: Many associations publish journals, reports, and newsletters that keep members informed on the latest developments and innovations in the field.
- Networking opportunities: Regular meetings, webinars, and online forums allow for engagement with peers and industry leaders, facilitating knowledge sharing and collaboration.
- Professional development: Many associations offer workshops, online courses, and certification programs designed to enhance the skills and credentials of their members.
- Job boards and career resources: They often maintain job boards specifically targeting computational science roles, making it easier to find opportunities tailored to oneās expertise.
For those serious about their professional growth, engaging with these associations is a strategic step forward.
Conferences and Workshops
Attending conferences and workshops in computational science is another strategic avenue for professional development. These events not only present cutting-edge research but also serve as excellent platforms for networking. Youāll find professionals from academia, industry, and government agencies congregating, exchanging ideas, and presenting their work.
Consider these key aspects of participating in such events:
- Hands-on learning: Workshops often include practical sessions where participants can gain experience with new tools and technologies that are shaping the field.
- Interdisciplinary exposure: Conferences often boast varied topics, bridging gaps between computational science and other disciplines. This can spark innovative ideas that may not have surfaced in a purely technical environment.
- Networking potential: Informal interactions during breaks or social events can lead to significant connections. A casual chat over coffee can lead to collaborative projects or job offers down the line.
- Visibility: Presenting research at these gatherings allows individuals to showcase their work and gain feedback. This not only builds confidence but also places one on the radar of influential professionals.
In summary, networking and professional growth are fundamental to thriving in computational science. Whether through associations or conferences, the relationships and knowledge gained can pave the way for both immediate opportunities and long-term career advancements.
Ending
In delving into the world of computational science, it becomes clear that this domain is both vast and intricate. As we draw together the insights gleaned from various sections of this article, a few critical elements stand out that are worth emphasizing. The significance of computational science lies not only in its methodological approaches and techniques but also in its growing relevance across multiple disciplines.
Computational science acts as a bridge, linking theoretical concepts with practical applications. This synergy fosters innovation and drives advancements in fields such as healthcare, climate modeling, and engineering. The ability to manipulate complex data sets and build predictive models is what sets computational scientists apart, making their roles essential in contemporary research and industry.
"To understand the power of computational science is to appreciate its role in shaping the future of our technology-driven world."
When we sift through the challenges ā from data management to ethical concerns ā it is equally important to recognize the opportunities that arise from tackling these hurdles. Organizations are increasingly on the lookout for skilled individuals who can bridge the gap between technology and analysis. Moreover, the career paths available are as diverse as the fields of study themselves, promising a spectrum of roles from academia to industry.
As we wrap this up, letās reflect on the key insights identifiedāskills in programming, an understanding of mathematics, and the ability to work effectively with data are paramount. The educational and professional development pathways outlined earlier pave a clear route for aspiring scientists. Thus, the takeaway here isn't merely about the current landscape but also about the future potential that computational science holds.
Recapping Key Insights
Here, I'll summarize some of the standout points discussed throughout the article:
- Diverse Career Paths: Opportunities abound in academia, industry, as well as government sectors.
- Essential Skills: Mastery in programming languages such as Python and C++, alongside solid mathematical and statistical knowledge, is critical.
- Educational Frameworks: Both undergraduate and graduate programs play a crucial role in shaping competent professionals.
- Future Growth: The job market is expected to grow steadily, reflecting the increasing reliance on computational methods in various disciplines.
Encouragement for Future Computational Scientists
To those stepping into this exciting arena, remember that every successful computational scientist started with a question. Your curiosity is your greatest asset; it will lead you toward unprecedented opportunities. Donāt shy away from challengesāinstead, view them as stepping stones to greater understanding and skill.
Begin with foundational knowledge, but allow your interests to guide you as you explore different niches within the field. Reach out to professional associations, attend workshops, and keep your network active. Engaging with existing professionals can provide invaluable insights and encourage growth.
Embrace continuous learning as an integral part of your journey. The tools and technologies in the realm of computational science evolve rapidly, thus, staying abreast of innovations will set you apart. Partnering theoretical knowledge with practical applications will empower you to make significant contributions to your chosen field.
In this endeavor, take heart; the world needs bright minds and innovative thinkers like you. The future of computational science is not just about understanding algorithms and models, but also about impacting society at large through your expertise. Your career might just be the key to unlocking future discoveries that could change our world.